12 Best Big Data Analytics Software Shortlist
After exhaustive research, I've curated a list of the 12 best big data analytics software to address your pressing data management challenges.
- RapidMiner - Best for streamlined data preparation
- Alteryx - Best for advanced data blending
- Cloudera - Best for scalable data storage
- Qlik - Best for associative data modeling
- SAS Big Data Analytics - Best for predictive analytics capabilities
- IBM Cloud Pak for Data - Best for AI-integrated data analysis
- MATLAB - Best for algorithm development
- Zoho Analytics - Best for business-driven data visualization
- Google Cloud BigQuery - Best for real-time analytics querying
- Azure Databricks - Best for Apache Spark integration
- Databricks Lakehouse Platform - Best for unified analytics architecture
- Tableau - Best for interactive data visualization
In the world of big data analytics software, Microsoft and other key players offer apps tailored for business intelligence and data analysis. Handling vast unstructured data, these tools, with their intuitive user interfaces, cater to data analysts, ensuring ease of use. They simplify data pipelines, making sense of metrics and natural language, whether on-premises or in a no-code environment.
With them, analyzing large amounts of data becomes straightforward, aiding swift business decisions. Business analytics, powered by the best data, has never been more accessible.
What is Big Data Analytics Software?
Big data analytics software is a powerful toolset designed to process, analyze, and interpret vast amounts of data, often too large for traditional databases to handle. These tools are commonly employed by data scientists, analysts, and business professionals to uncover hidden patterns, correlations, and insights within massive datasets.
By doing so, they enable industries ranging from healthcare to finance to retail to make informed decisions, predict future trends, and develop data-driven strategies that can offer a competitive edge.
Overviews of the 12 Best Big Data Analytics Software
1. RapidMiner - Best for streamlined data preparation
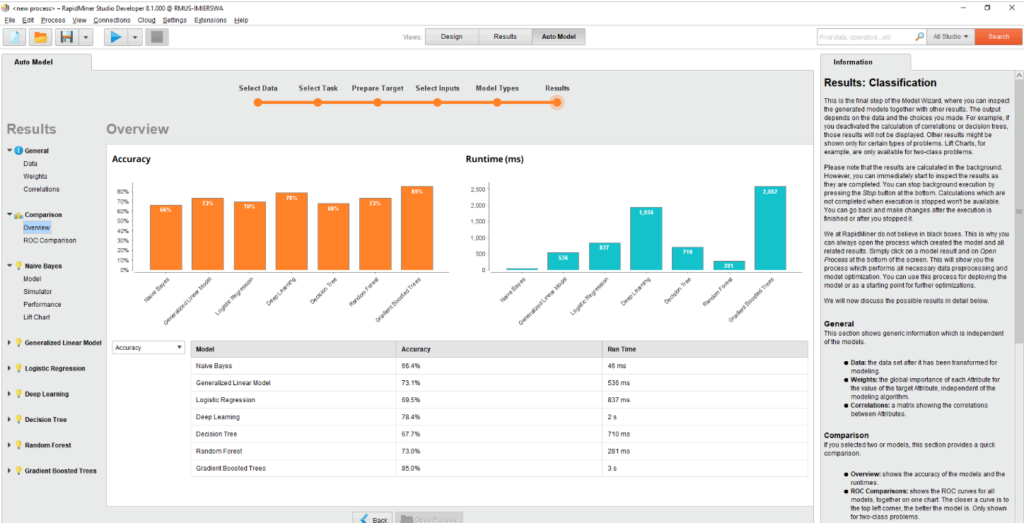
RapidMiner, a leading data science platform, excels in simplifying the often complicated steps of data preparation. By focusing on a user-friendly experience, it offers a comprehensive solution that caters to both novices and experts in the field of data analytics.
Why I Picked RapidMiner:
From the myriad of tools I've encountered, RapidMiner consistently stood out due to its robust and intuitive platform, tailored to make data preparation smoother. Its dedication to streamlining this phase, which is often the most time-consuming in the analytics process, is evident.
This efficiency is why I firmly believe RapidMiner is the best for anyone keen on simplifying their data preparation journey.
Standout Features & Integrations:
RapidMiner’s platform provides tools for data cleansing, transformation, and enrichment. Its visual workflow designer is particularly notable, allowing users to quickly map out their data processes. On the integration front, it supports a plethora of databases, applications, and file formats, ensuring flexibility in sourcing and connecting data.
Pricing:
From $15/user/month (billed annually)
Pros:
- Comprehensive data preprocessing tools
- Visual workflow design capabilities
- Wide range of integration options
Cons:
- The steeper learning curve for beginners
- Annual billing can lock in users
- Limited advanced features in the basic plan
2. Alteryx - Best for advanced data blending
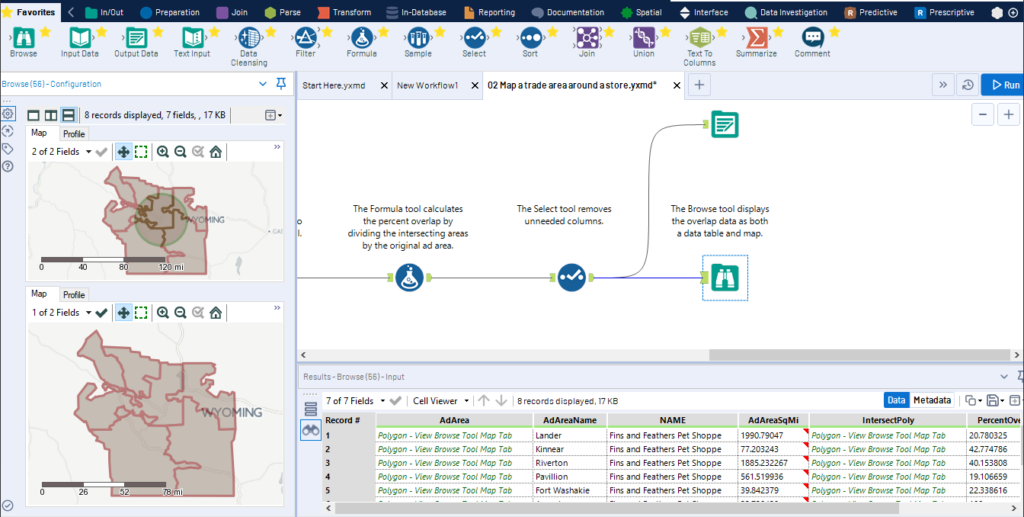
Alteryx is a renowned platform that emphasizes the power and precision of data blending and analytics. Through its robust toolkit, it offers users an exceptional ability to combine diverse datasets, preparing them for detailed analysis.
Why I Picked Alteryx:
Among the array of platforms I reviewed, Alteryx rose above its peers with its sophisticated yet user-centric approach to data blending. The software offers precise controls, enabling customization while merging disparate data sources.
Given its unique prowess in this domain, I am convinced Alteryx is the top choice for those seeking advanced data blending capabilities.
Standout Features & Integrations:
Alteryx boasts features like spatial and predictive analytics, alongside its core data blending tools. Furthermore, its drag-and-drop interface provides an intuitive experience. Integration-wise, Alteryx collaborates with major data platforms like Tableau, SQL, and more.
Pricing:
From $45/user/month (billed annually)
Pros:
- Precision in data blending tasks
- Integrates well with other analytics platforms
- Predictive and spatial analytics capabilities
Cons:
- May be overkill for simpler tasks
- Premium pricing for advanced features
- Requires some learning for best use
3. Cloudera - Best for scalable data storage
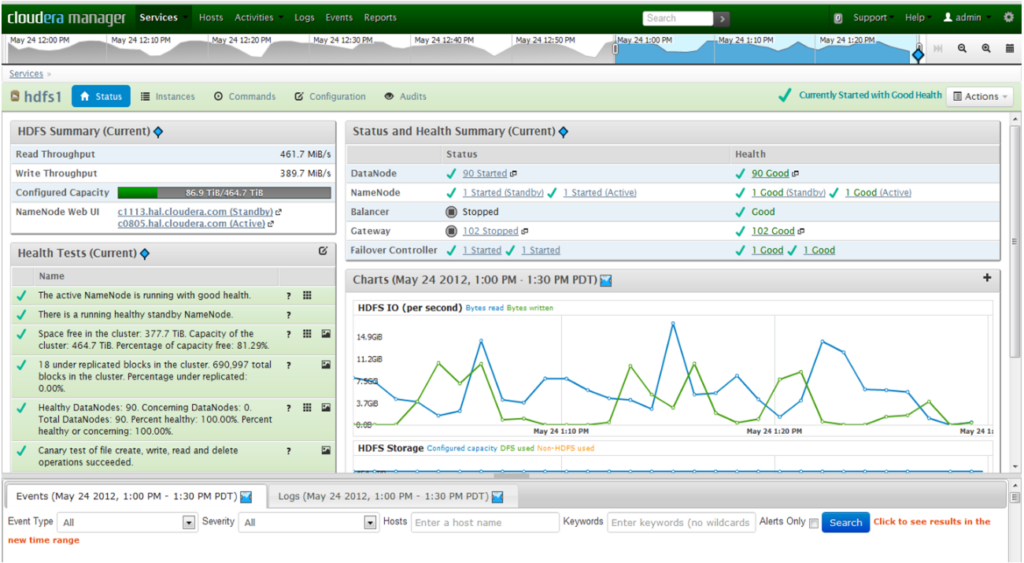
Cloudera stands out as a reliable platform dedicated to offering scalable solutions for data storage and management. It's designed to grow with your business, ensuring that as your data needs expand, the system can adapt effortlessly.
Why I Picked Cloudera:
When I was determining the top tools in scalable data storage, Cloudera repeatedly made an impression. It's not just about the amount of data it can handle; it's about how it effectively manages and processes it. Given its stellar reputation and performance in scalability, I hold the view that Cloudera is the go-to for businesses anticipating exponential data growth.
Standout Features & Integrations:
Cloudera offers features like data warehousing, machine learning capabilities, and real-time analytics. Importantly, it supports integration with a variety of open-source tools and platforms, ensuring compatibility and extensibility.
Pricing:
Pricing upon request
Pros:
- Robust data warehousing capabilities
- Machine learning integrations
- Wide range of supported open-source tools
Cons:
- Can be complex for beginners
- Licensing can be intricate for some users
- Deployment might require technical expertise
4. Qlik - Best for associative data modeling
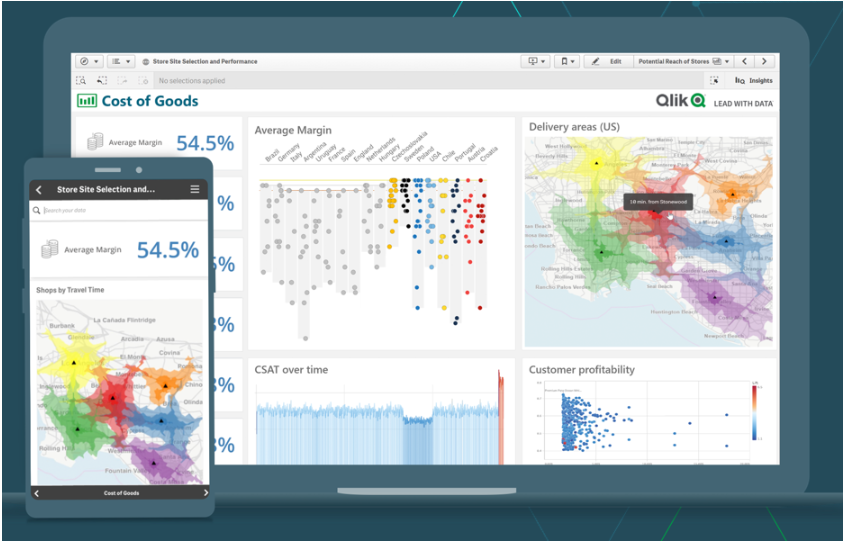
With Qlik, users have a tool that excels in associative data modeling, allowing for deeper insights by connecting related data points. Its unique engine facilitates understanding complex relations within datasets, driving smarter decisions.
Why I Picked Qlik:
Selecting tools that truly shine in associative data modeling is no simple feat. Yet, my assessment of Qlik confirmed its reputation as a leader in this realm. The depth it offers in analyzing intricate data relations is unparalleled. This makes me believe that for those seeking to explore how data points interconnect, Qlik is undeniably the best choice.
Standout Features & Integrations:
Qlik's associative engine is its star feature, allowing for dynamic data visualization. Additionally, it offers robust data integration capabilities. With Qlik, users can integrate with multiple data sources, from SQL databases to cloud platforms.
Pricing:
From $30/user/month (billed annually)
Pros:
- Dynamic associative data modeling
- Flexible data integration options
- User-friendly visualization tools
Cons:
- Some advanced features behind premium paywalls
- Mobile experience could be improved
- Might be over-comprehensive for small projects
5. SAS Big Data Analytics - Best for predictive analytics capabilities
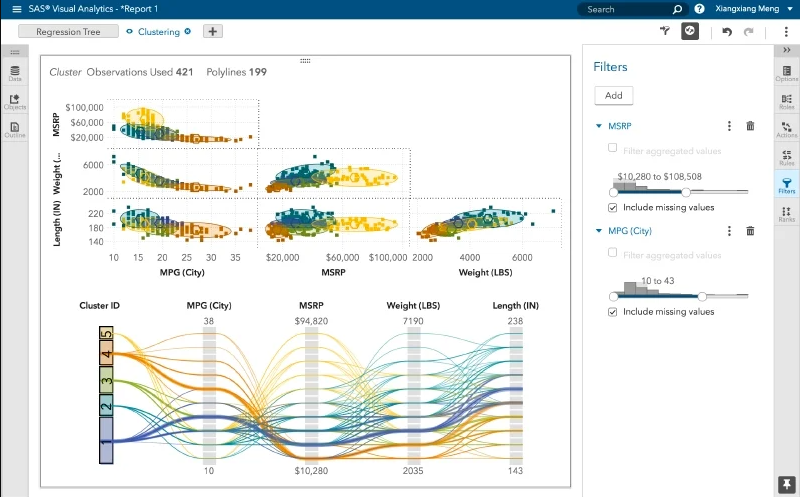
SAS Big Data Analytics is a powerful solution that delves deep into datasets, leveraging them to forecast future trends and patterns. Its strength in predictive analytics sets it apart, enabling businesses to proactively strategize based on projected insights.
Why I Picked SAS Big Data Analytics:
During my review, choosing a tool with strong predictive prowess became imperative, and SAS consistently emerged as a leader. The depth of its predictive analytics, bolstered by decades of refinement, is truly remarkable.
In my opinion, for those looking to tap into future insights using their current data, SAS Big Data Analytics is undoubtedly the optimal choice.
Standout Features & Integrations:
The platform comes equipped with advanced analytics algorithms, visual data exploration, and automated machine learning. Its integrative capacity is commendable, with compatibility spanning across multiple data sources and formats, including cloud-based databases and real-time data streams.
Pricing:
Pricing upon request
Pros:
- Industry-leading predictive analytics
- Wide range of integrative capacities
- Comprehensive visual exploration tools
Cons:
- Might have a steeper learning curve for beginners
- Licensing and pricing can be complex
- Requires considerable setup and configuration
6. IBM Cloud Pak for Data - Best for AI-integrated data analysis
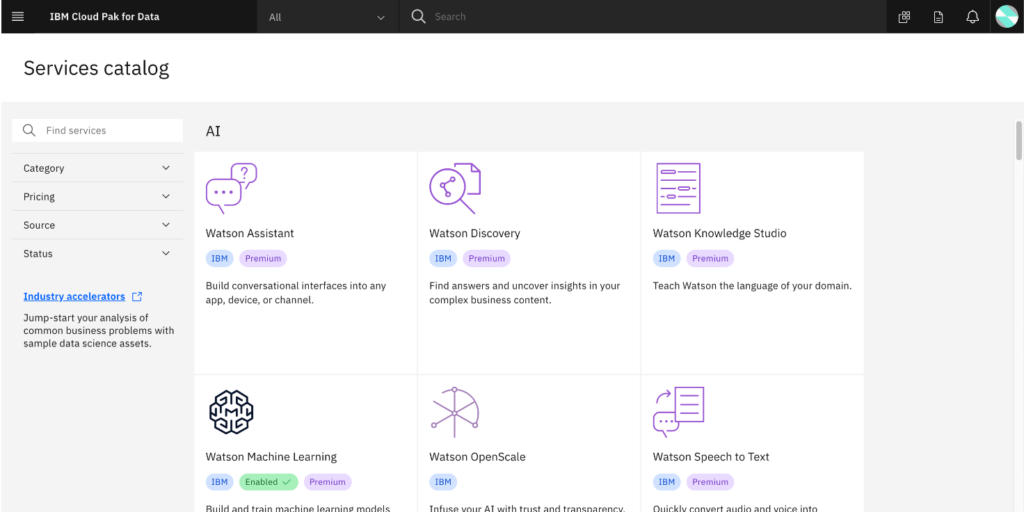
IBM Cloud Pak for Data is a unified data and AI platform, that integrates diverse analytics tools to derive intelligence from data. Its infusion of AI into data analysis makes it a standout choice for businesses aiming to supercharge their analytics.
Why I Picked IBM Cloud Pak for Data:
In selecting an AI-driven analytics platform, IBM's solution came forward as a distinguished contender. The manner in which it integrates AI into its data workflows, facilitating more nuanced analyses, genuinely resonated with me.
Given this capability, I am of the view that IBM Cloud Pak for Data is the premier choice for businesses yearning for AI-enhanced analytical power.
Standout Features & Integrations:
IBM Cloud Pak for Data offers features like data virtualization, knowledge cataloging, and AI-driven insights. Additionally, it integrates with Red Hat OpenShift, Watson AI, and a plethora of databases and data lakes.
Pricing:
From $95/user/month (billed annually)
Pros:
- Robust AI-driven analytical features
- Comprehensive data virtualization capabilities
- Integration with leading IBM tools and services
Cons:
- Might be over-comprehensive for small-scale projects
- A high starting price point for some businesses
- Dependency on the IBM ecosystem
7. MATLAB - Best for algorithm development
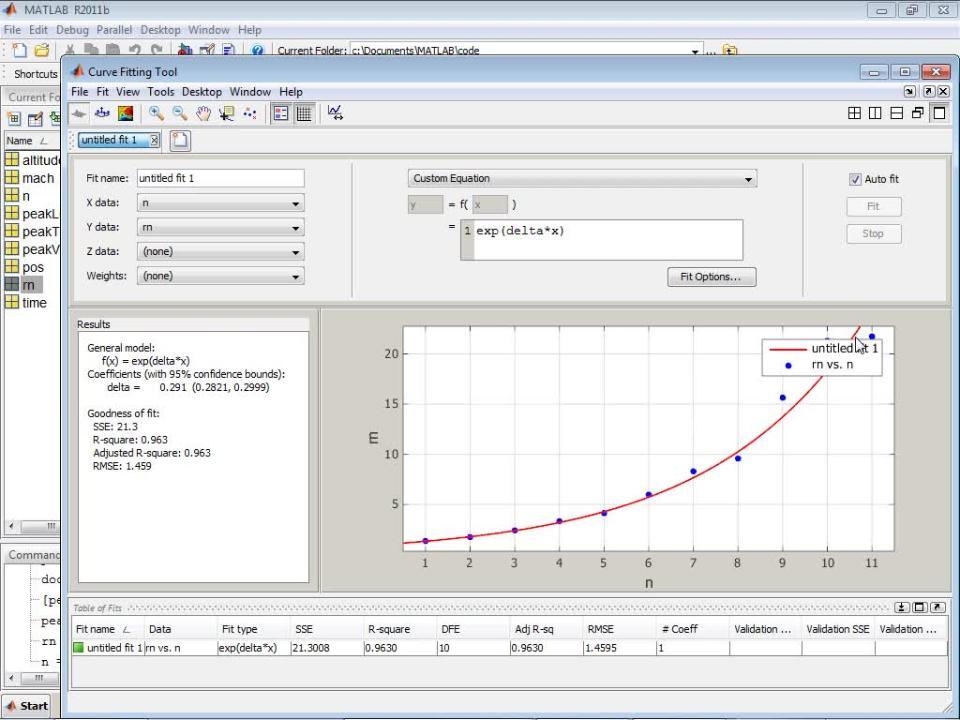
MATLAB, renowned in the scientific and engineering communities, offers a high-performance environment for developing complex algorithms. Its distinct proficiency in algorithm creation and validation ensures that users can develop precise, efficient solutions.
Why I Picked MATLAB:
My task of determining a leading tool for algorithm development continually circled back to MATLAB. Its long-standing reputation, coupled with its extensive toolbox for algorithm creation, played a pivotal role in my judgment.
Based on this, I firmly believe MATLAB is the foremost choice for professionals focused on intricate algorithm development.
Standout Features & Integrations:
MATLAB thrives on its robust toolboxes for signal processing, machine learning, and computational finance. Integration-wise, it collaborates effortlessly with C/C++, Java, .NET, and Python, allowing for a more expansive utility.
Pricing:
From $49/user/month (billed annually)
Pros:
- Exceptional algorithm development and testing tools
- Integration capabilities with major programming languages
- Comprehensive toolboxes for specialized tasks
Cons:
- Can be complex for beginners
- Premium pricing for specialized toolboxes
- Requires consistent updates for toolboxes and add-ons
8. Zoho Analytics - Best for business-driven data visualization
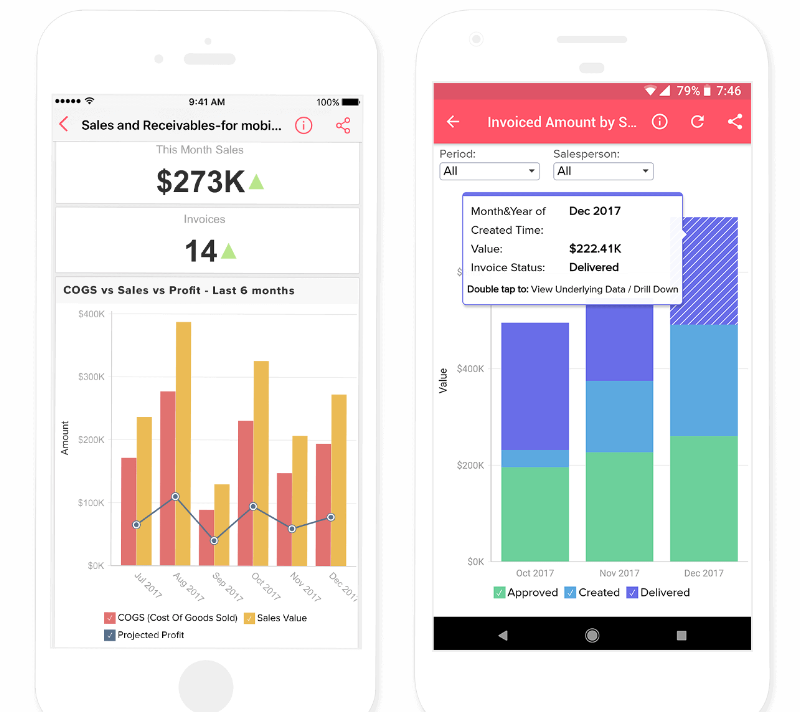
Zoho Analytics provides an intuitive platform that businesses can use to derive insights from their data. Its ability to present data in visually compelling formats makes it a crucial tool for businesses aiming to make data-driven decisions rooted in clear visual representation.
Why I Picked Zoho Analytics:
My journey in selecting a data visualization tool directed me towards Zoho Analytics primarily due to its straightforward interface paired with powerful visualization capabilities. What truly set it apart in my evaluation was its dedication to facilitating business-oriented data interpretation.
For firms looking to transform raw data into easily digestible visual insights, Zoho Analytics is a paramount choice.
Standout Features & Integrations:
Key features of Zoho Analytics include drag-and-drop functionality, geo-visualization capabilities, and pivot tables. It integrates smoothly with multiple Zoho Suite products, such as Zoho CRM, as well as external platforms like Google Ads and Salesforce.
Pricing:
From $22/user/month (billed annually)
Pros:
- User-friendly interface
- Rich set of visualization tools
- Extensive integration options
Cons:
- Can be overwhelming for absolute beginners
- Advanced features require a steeper learning curve
- Some integrations may require manual configuration
9. Google Cloud BigQuery - Best for real-time analytics querying
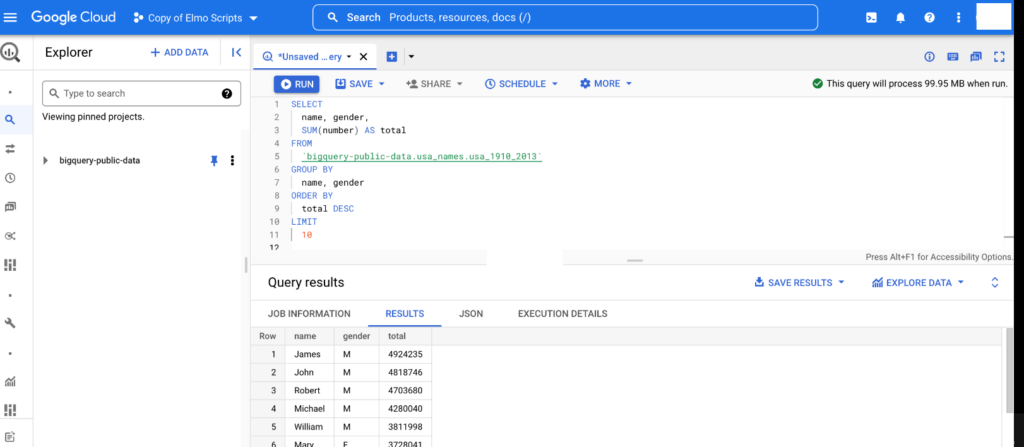
Google Cloud BigQuery is a fully managed data warehouse solution that provides super-fast SQL analytics across large datasets. Its efficiency in handling real-time analytics queries ensures that businesses can acquire instant insights without any significant delay.
Why I Picked Google Cloud BigQuery:
The quest for a robust real-time analytics solution invariably led me to Google Cloud BigQuery. Its prowess in handling immense volumes of data and delivering immediate results made a significant impression.
In my estimation, when it comes to obtaining insights in real-time from vast datasets, BigQuery stands unmatched.
Standout Features & Integrations:
BigQuery boasts features like automatic backup and easy restore, machine learning integration, and geospatial analysis. It also offers integration with various Google Cloud services and supports multiple third-party tools and services.
Pricing:
Starting from $20/TB (based on the volume of queries processed)
Pros:
- Scalability without the need for additional infrastructure
- Built-in machine learning capabilities
- Comprehensive security features
Cons:
- The pricing model can be complex for new users
- Requires familiarity with SQL for optimal usage
- Limited built-in data visualization tools
10. Azure Databricks - Best for Apache Spark integration
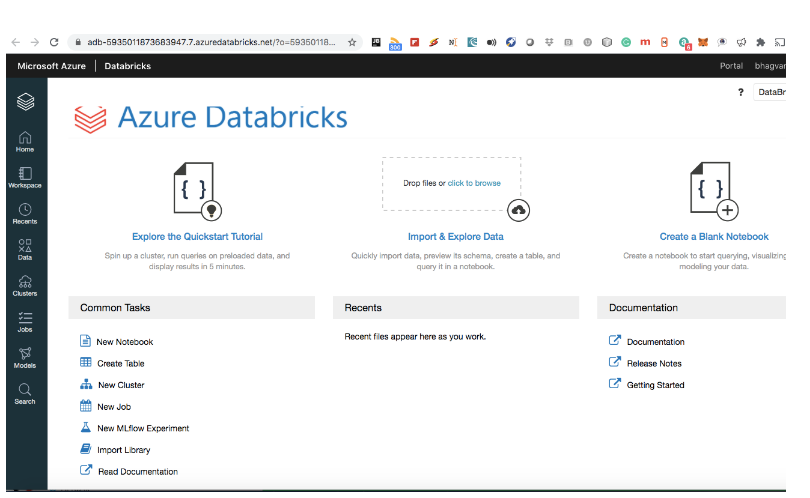
Azure Databricks offers a unified analytics platform, optimized for Azure, that brings together big data and artificial intelligence. With its impeccable integration capabilities with Apache Spark, it provides a streamlined environment for processing vast datasets.
Why I Picked Azure Databricks:
In seeking a platform with top-tier Apache Spark integration, Azure Databricks was a clear standout. The synergy between Azure and Apache Spark, combined with the platform's efficiency, shaped my decision. For professionals leaning heavily on Apache Spark for their data processing needs, I contend that Azure Databricks is the prime choice.
Standout Features & Integrations:
Azure Databricks shines with features like collaborative notebooks, real-time workspace, and enterprise-grade security. It boasts tight-knit integration with Azure services such as Azure Blob Storage, Azure Data Factory, and Azure SQL Data Warehouse.
Pricing:
From $0.20/DBU (Databricks compute unit) per hour
Pros:
- Smooth integration with Apache Spark
- Collaboration-friendly platform
- Tight security measures
Cons:
- Pricing can be challenging to gauge without careful analysis
- Might have a learning curve for those new to Databricks or Azure
- Integration with non-Azure services can require extra configurations
11. Databricks Lakehouse Platform - Best for unified analytics architecture
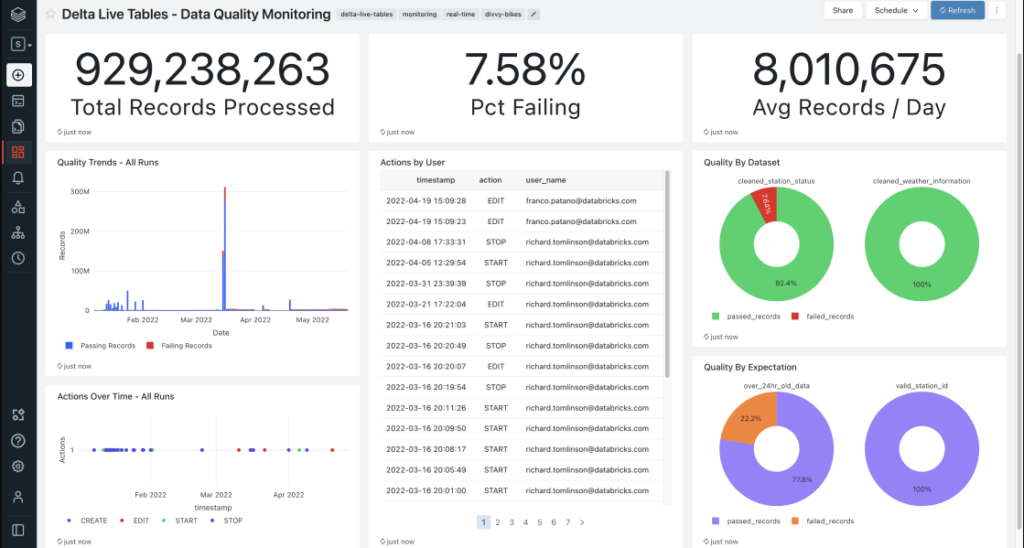
Databricks Lakehouse Platform blends the best attributes of data lakes and data warehouses, ensuring an analytical experience. With its emphasis on bridging the divide between big data and traditional analytics, the Lakehouse architecture is a revolutionary step in unified data processing and analytics.
Why I Picked Databricks Lakehouse Platform:
My decision to select the Databricks Lakehouse Platform stemmed from its innovative approach to analytics. In the realm of data management, it's uncommon to find a platform that can truly unify disparate architectures. Databricks Lakehouse Platform, in my judgment, is at the forefront of redefining how businesses perceive and leverage analytics architecture.
Standout Features & Integrations:
Databricks Lakehouse features include ACID transactions, schema enforcement, and storage optimization, ensuring both reliability and performance. Integration-wise, it connects smoothly with most big data tools and platforms, such as Apache Spark, Delta Lake, and MLflow.
Pricing:
Pricing upon request
Pros:
- A holistic approach to data analytics
- Combines benefits of data lakes and data warehouses
- High scalability and performance
Cons:
- Might pose a steep learning curve for some users
- Reliance on other Databricks tools may be necessary for full utilization
- Customized solutions can be costly
12. Tableau - Best for interactive data visualization
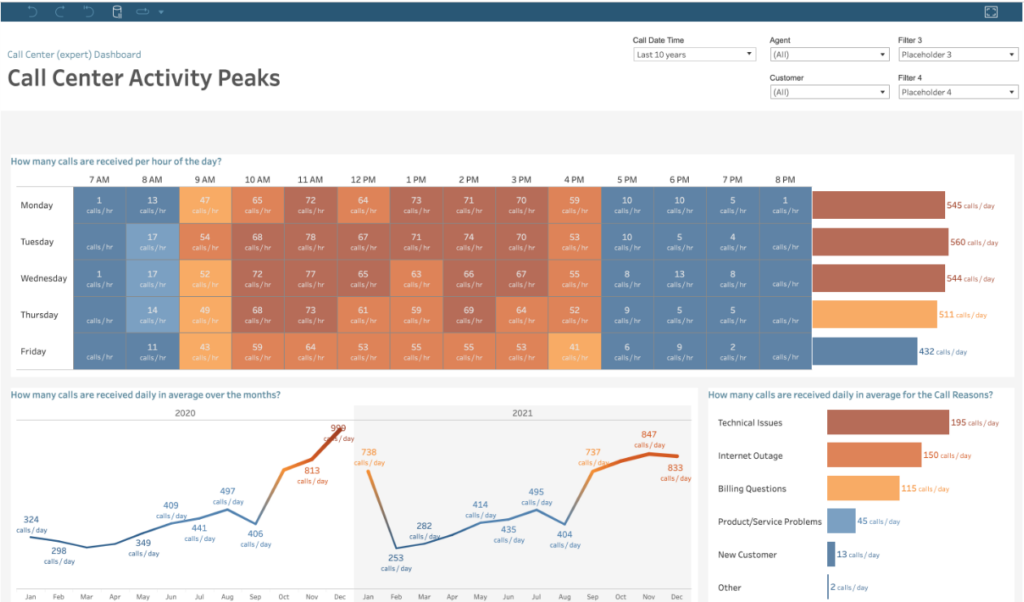
Tableau stands as a leading tool in the data visualization space, empowering users to transform raw data into insightful, interactive visualizations. Its capacity to make complex data patterns understandable through visual interactivity is what sets it apart in the analytics world.
Why I Picked Tableau:
After exploring numerous visualization tools, my choice gravitated towards Tableau primarily due to its unmatched interactivity. The tool's ability to convey complex data stories in a visually compelling and interactive manner made it distinct. For those aiming to harness the power of interactive visualization, Tableau is, in my opinion, a top contender.
Standout Features & Integrations:
Tableau's most notable features are its drag-and-drop interface, real-time data analytics, and a robust set of visualization tools. It integrates effectively with a wide range of data sources, from spreadsheets to cloud services, including but not limited to Google Analytics, SQL databases, and Amazon Web Services.
Pricing:
From $70/user/month (billed annually)
Pros:
- Advanced yet user-friendly visualization capabilities
- Extensive data source integration options
- Collaborative dashboard sharing
Cons:
- Requires training for advanced functionalities
- Pricing can be prohibitive for smaller teams
- Some performance issues with very large datasets
Other Notable Big Data Analytics Software
Below is a list of additional big data analytics software that I shortlisted, but did not make it to the top 12. Definitely worth checking them out.
- Azure Data Lake Analytics - Good for scalable big data processing
- Azure Synapse Analytics - Good for combining big data and data warehousing
- Confluent - Good for Apache Kafka-based streaming
- Apache Hadoop - Good for distributed storage and processing
- Starburst - Good for querying diverse data sources
- IBM Watson Analytics - Good for AI-driven data insights
- Apache Spark - Good for in-memory data analytics
- Splunk - Good for operational intelligence from machine data
- Teradata Vantage - Good for high-performance analytics at scale
- Hortonworks - Good for open-source data platform solutions
- dbt - Good for analytics engineering workflows
- KNIME - Good for visual data science workflows
Selection Criteria For Choosing the Best Big Data Analytics Software
Having spent a considerable amount of time diving into the world of big data analytics tools, I've tested and evaluated numerous software options available in the market. My pursuit was driven by a need for robust functionality, specific features tailored to handling vast amounts of data, and an interface that aligns with modern user expectations.
From more than 30 tools I explored, only a select few truly stood out, and here's why:
Core Functionality
- Data Ingestion: Ability to intake data from multiple sources efficiently.
- Data Processing: Handle, clean, and prepare large datasets for analysis.
- Scalability: Ensure performance even as data grows in magnitude.
- Distributed Computing: Capability to process data across multiple nodes or servers.
- Advanced Analytics: Offering statistical analysis, predictive modeling, and machine learning capabilities.
Key Features
- Interactive Visualizations: Offers visualization tools that allow users to manipulate and interact with their data graphs and charts.
- Real-time Processing: The ability to analyze data in real-time, is crucial for industries where timely insights make a difference.
- Data Storage: A system in place for securely storing and retrieving large datasets.
- Security Protocols: Comprehensive security measures, including encryption and role-based access controls.
- API Integration: Facilitating connection with other tools or platforms for enhanced functionality.
Usability
- Intuitive Design: An interface that is straightforward, minimizing the learning curve for new users.
- Dashboard Customizability: Allows users to tailor their workspace to show the most relevant data and insights.
- Role-Based Access: Ensure that different teams or members can access only the specific data they need, enhancing security.
- Support & Documentation: Availability of extensive user guides, tutorials, and responsive customer support to aid in onboarding and troubleshooting.
- Platform Compatibility: Software should be accessible across devices, whether it's a desktop, tablet, or mobile.
Most Common Questions Regarding Big Data Analytics Software
What are the benefits of using big data analytics software?
Using big data analytics software offers several advantages:
- Data-Driven Decision Making: It provides insights derived from large datasets, enabling businesses to make informed decisions.
- Predictive Analysis: Companies can forecast trends and patterns, helping them stay ahead in the market.
- Enhanced Efficiency: Automation of data processing tasks leads to streamlined operations and reduced manual labor.
- Improved Customer Experience: By understanding customer behavior and patterns, businesses can offer personalized experiences.
- Risk Management: By analyzing vast amounts of data, companies can identify potential risks and strategize accordingly.
How much do these tools typically cost?
The cost of big data analytics software can vary greatly depending on the tool, its features, and the scale of operations. Typically, prices can range anywhere from $10/user/month to over $1,000/user/month.
What are the different pricing models for big data analytics tools?
There are several pricing models for big data analytics software:
- Subscription-Based: Monthly or yearly recurring fees.
- Pay-as-you-go: Pricing is based on the volume of data processed or stored.
- Freemium: Offers basic features for free, with a fee for advanced features.
- One-time License: Pay a one-time fee and own the software for life.
What is the typical range of pricing for these tools?
While there's a vast range in pricing, most big data analytics tools fall within the $50 to $500/user/month range, with enterprise-level solutions leaning towards the higher end.
Which is the cheapest big data analytics software?
While the definition of "cheapest" can vary based on features and capabilities offered, many tools like Apache Hadoop and Apache Spark provide robust capabilities with no licensing fees, though there may be costs associated with setup, maintenance, and scaling.
Which is the most expensive big data analytics software?
Enterprise solutions like Teradata Vantage and some configurations of IBM Watson Analytics tend to be on the pricier side, with costs often exceeding $1,000/user/month, especially when considering additional features and large-scale operations.
Are there any free big data analytics tools?
Yes, there are several free options available. Apache Hadoop and Apache Spark are popular open-source tools. However, it's important to note that while the software might be free, there could be costs associated with infrastructure, maintenance, or additional modules.
Why is there such a significant price difference among these tools?
The price difference often stems from the scale of operations the tool is designed for, the features it offers, the quality of customer support, and the brand's reputation. Enterprise-level solutions with extensive features, integrations, and top-tier support will generally cost more than basic tools tailored for smaller businesses or specific tasks.
Other Data Management Software Reviews
Summary
Choosing the right big data analytics software is crucial for deriving actionable insights from vast datasets. While numerous tools exist in the market, it's essential to pinpoint one that aligns with your specific needs and budget.
Key Takeaways
- Understand your needs: Before diving into the plethora of options, outline what you're hoping to achieve with the software. Whether it's predictive analysis, improved customer experiences, or streamlined operations, defining clear objectives will narrow down your choices.
- Consider the total cost: While pricing models vary—ranging from subscription-based to pay-as-you-go—it's important to account for potential hidden costs. This includes infrastructure, maintenance, training, and scalability.
- Usability matters: Even the most feature-rich software can fall short if it isn't user-friendly. Prioritize tools that offer an intuitive interface, robust customer support, and efficient onboarding processes to ensure your team can leverage its full potential.
Want More?
While this guide aims to provide comprehensive insights into the best big data analytics software, the tech landscape is vast and ever-evolving. There are endless resources, including big data podcasts, books, and more, to further your learning journey.
You can also join The CTO Club mailing list for more resource round-ups, tool reviews, and expert insights.