12 Best Big Data Software Shortlist
After a thorough evaluation, I've hand-picked the 12 best big data software, chosen precisely to address your concerns.
- Elasticsearch - Best for real-time search and analytics
- MATLAB - Best for mathematical computing with big data
- Qubole - Best for scalable cloud-native big data analytics
- OneTrust - Best for data discovery and compliance
- Explorium - Best for external data platform integration
- Tableau - Best for interactive big data visualization
- Microsoft SQL Server - Best for relational database management
- Google Cloud BigQuery - Best for serverless and highly-scalable data warehousing
- Apache Flink - Best for real-time data processing and analytics
- Apache Kafka - Best for real-time data streaming platforms
- Apache Spark - Best for large-scale data processing tasks
- IBM Big data analytics - Best for enterprise-level data analysis and insights
In the sophisticated world of big data software, apps, and analytics platforms stand out, offering businesses unparalleled insights. Big data analytics tools empower data scientists to turn large data sets into actionable business intelligence. Whether it's the complexities of programming languages, on-premise solutions on Windows, or no-code web services, there are tools that make data transformation and predictive modeling feasible.
Data quality is paramount, and the right big data software simplifies this, streamlining business analytics and ensuring accurate predictive analysis from trusted source data. Trust in these tools; they're the future of business insights.
What Is a Big Data Software?
Big data software refers to a suite of tools and platforms designed to manage, process, and analyze vast sets of complex data that traditional data processing systems cannot handle. Businesses, researchers, and governments employ these software tools to extract insights, inform decision-making processes, and identify patterns or trends within the data.
Industries ranging from healthcare to finance leverage big data technologies to improve operations, tailor marketing strategies, predict consumer behavior, and drive innovations in their respective fields.
Overviews of the 12 Best Big Data Software
1. Elasticsearch - Best for real-time search and analytics
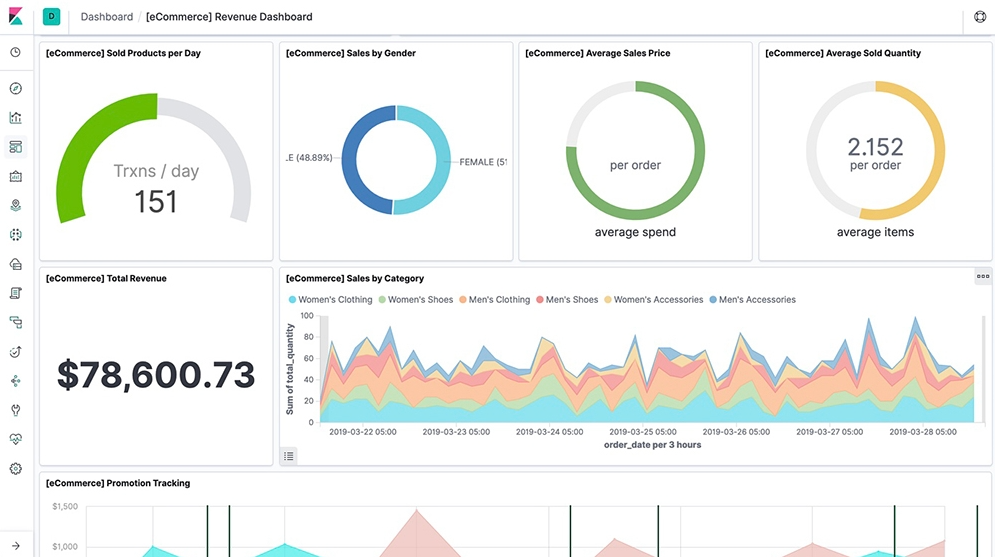
Elasticsearch is an open-source, search, and analytics engine that allows for real-time data exploration and visualization. With the capability to handle large volumes of data on the fly, it facilitates quick insights into complex datasets, making it ideal for real-time search and analytics.
Why I Picked Elasticsearch:
I chose Elasticsearch for this list because of its exceptional ability to deliver real-time analytics, its robustness, and its extensive community support. In comparing various tools, Elasticsearch stood out for its integration with diverse data types and its flexible querying options.
I determined that it's best for real-time search and analytics due to its fast indexing and search capabilities, allowing users to access insights instantly.
Standout Features & Integrations:
Elasticsearch excels in full-text search capabilities, and its distributed nature makes it highly scalable. Its real-time indexing allows for instantaneous data processing and insight extraction. Important integrations include those with Kibana for visualization, Logstash for data collection, and Beats for data shipment, forming a robust Elastic Stack.
Pricing:
From $7/user/month (billed annually)
Pros:
- Open-source with a strong community
- Extensive analytics capabilities
- Scalable and adaptable to various business needs
Cons:
- Steeper learning curve for beginners
- May require substantial hardware resources for extensive datasets
- Complexity in managing and configuring the system
2. MATLAB - Best for mathematical computing with big data
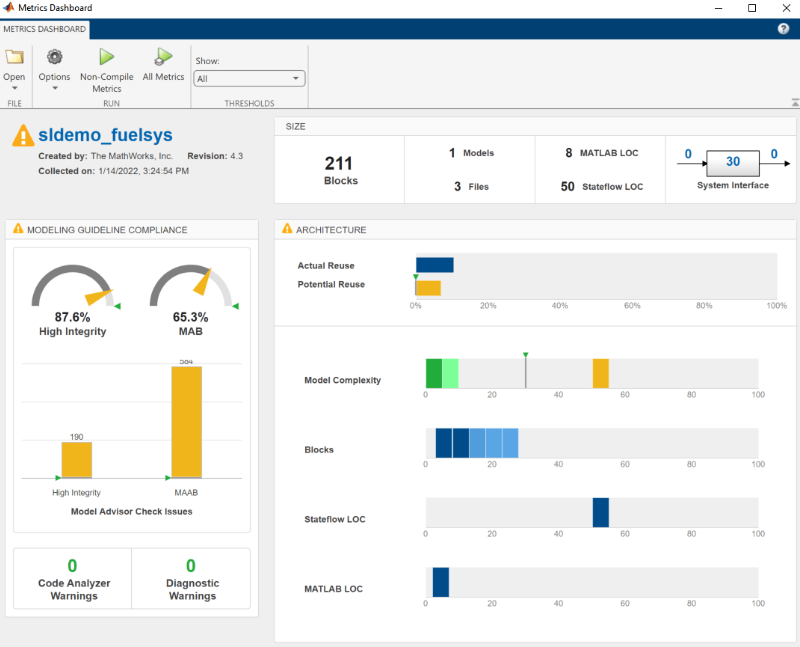
MATLAB, produced by MathWorks, is a high-performance language for technical computing. Geared towards functions like algorithm development, data visualization, and numerical computation, its design is prime for those seeking powerful mathematical computing in the realm of big data.
Why I Picked MATLAB:
When I dove into the world of big data software, choosing MATLAB was inevitable. Its reputation in the scientific and engineering communities precedes it. The sheer power it brings to mathematical computations, especially when handling big data, distinguishes it.
In my opinion, when comparing various tools, MATLAB firmly stands as the best for complex mathematical operations within the big data environment.
Standout Features & Integrations:
MATLAB is renowned for its toolbox, which contains a vast array of functions for signal processing, machine learning, and more. Its Simulink feature allows for model-based designs, enhancing the simulation and model-testing process. MATLAB integrates with many third-party environments and tools, including C/C++, Java, .NET, and Python, ensuring smooth workflows across different platforms.
Pricing:
From $50/user/month (billed annually)
Pros:
- Extensive toolbox with diverse functionalities
- Facilitates both basic and advanced mathematical computations
- Robust integration capabilities with other languages and platforms
Cons:
- Might be overkill for simpler tasks
- Relatively steep learning curve for those unfamiliar with its language
- Licensing structure can be complex for larger teams
3. Qubole - Best for scalable cloud-native big data analytics
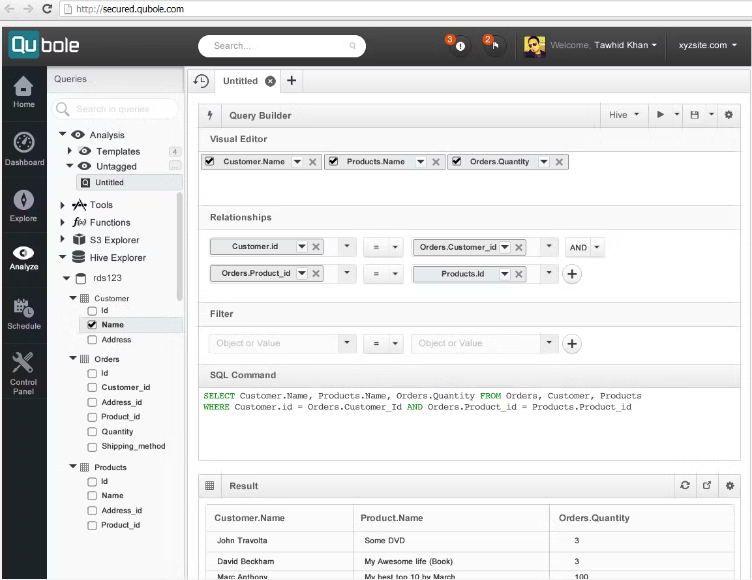
Qubole is a cloud-native big data platform designed to simplify the management of your data processing infrastructure. It specializes in facilitating scalable big data analytics, ensuring companies can manage vast amounts of information efficiently, in line with its claim of being best for such tasks.
Why I Picked Qubole:
In my journey of assessing big data tools, Qubole emerged as a compelling choice. Its inherent ability to scale based on workload and its adaptability in cloud environments makes it a standout. Comparing its features with others, I determined that Qubole truly shines when it comes to scalable, cloud-native big data analytics.
Standout Features & Integrations:
One of Qubole's strengths is its autonomous data platform that allows for self-managing and self-optimizing tasks, ensuring that resources are used efficiently. Its multi-cloud support means businesses aren't restricted to one provider.
Integration-wise, Qubole smoothly connects with a plethora of data stores, BI tools, and major cloud platforms including AWS, Azure, and Google Cloud, reinforcing its cloud-native essence.
Pricing:
From $40/user/month (billed annually)
Pros:
- Autonomous features reduce administrative overhead
- Versatility with multi-cloud support
- Robust integrations with key BI tools and data storage solutions
Cons:
- Might present a learning curve for those new to cloud-native platforms
- Pricing can become steep for extensive usage
- Some features might be excessive for smaller data tasks
4. OneTrust - Best for data discovery and compliance
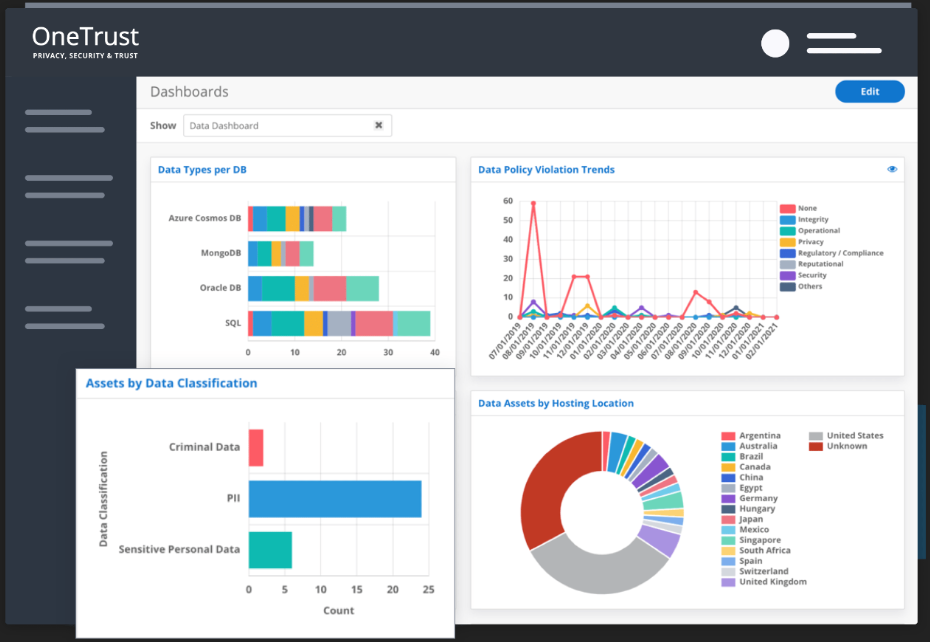
OneTrust provides a platform focused on data discovery, privacy, security, and third-party risk. In today's stringent regulatory environment, its commitment to ensuring data compliance stands tall, thus validating its positioning as the best tool for data discovery and compliance.
Why I Picked OneTrust:
Navigating the crowded space of data management tools, OneTrust immediately captured my attention. The tool's dedication to integrating both data discovery and compliance resonated with the emerging challenges of modern businesses.
Through meticulous comparison and judgment, I discerned that OneTrust offers an unmatched equilibrium between discovering hidden data and ensuring its compliance.
Standout Features & Integrations:
OneTrust boasts features like automated data discovery, a central repository for all data-related assets, and assessment automation which are pivotal for businesses dealing with intricate data ecosystems.
On the integration front, OneTrust collaborates effortlessly with various enterprise tools and platforms, making it a cinch for businesses to weave into their existing infrastructures.
Pricing:
From $50/user/month (billed annually)
Pros:
- Comprehensive data discovery mechanisms
- Emphasis on compliance aligns with global regulations
- Wide-ranging integrations with major enterprise tools
Cons:
- Can be complex for smaller organizations
- Requires a level of expertise for optimal use
- The multitude of features might be overwhelming for new users
5. Explorium - Best for external data platform integration
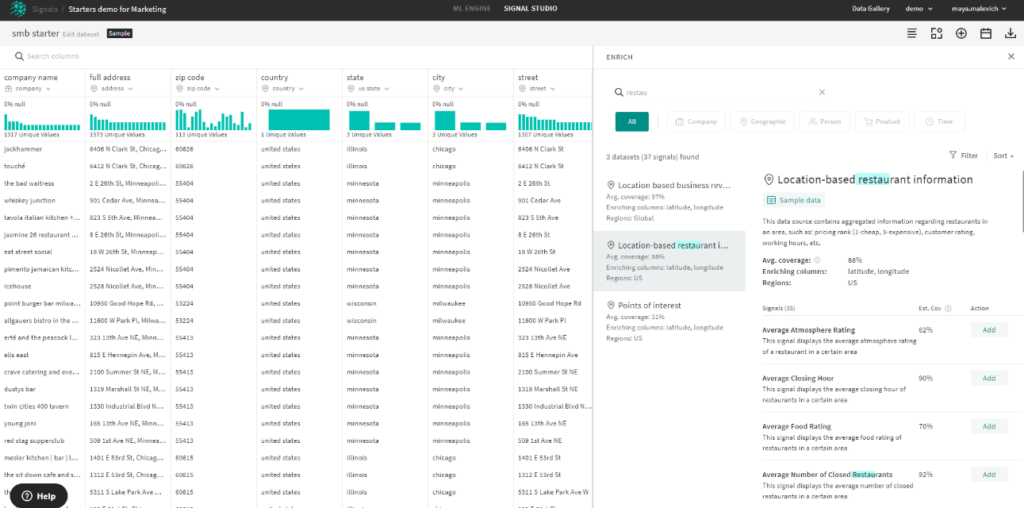
Explorium is a cutting-edge platform that empowers companies to integrate external data easily into their analytics and machine learning models. Given the increasing importance of leveraging external data sources, Explorium's specialized focus makes it the premier choice for external data platform integration.
Why I Picked Explorium:
The vast landscape of data platforms often left me yearning for a tool that effortlessly bridges the gap between an organization's internal data and the vastness of external data sources. Upon close inspection and comparison, Explorium emerged as a clear front-runner.
The platform's commitment to fostering easy integration of external data sources reaffirmed its position as the best for this use case.
Standout Features & Integrations:
At its core, Explorium thrives by enabling businesses to enrich their existing data with a myriad of external sources. It’s a dynamic data discovery and AI-driven insights pave the way for more informed decisions. Furthermore, its compatibility with popular data processing and storage platforms ensures businesses can integrate it without uprooting their current ecosystems.
Pricing:
From $60/user/month (billed annually)
Pros:
- Enables access to a vast array of external data sources
- AI-driven insights improve data comprehension
- Robust compatibility with popular data platforms
Cons:
- Initial setup may require a learning curve
- Might be overkill for businesses with limited external data needs
- Custom integrations can be resource-intensive
6. Tableau - Best for interactive big data visualization
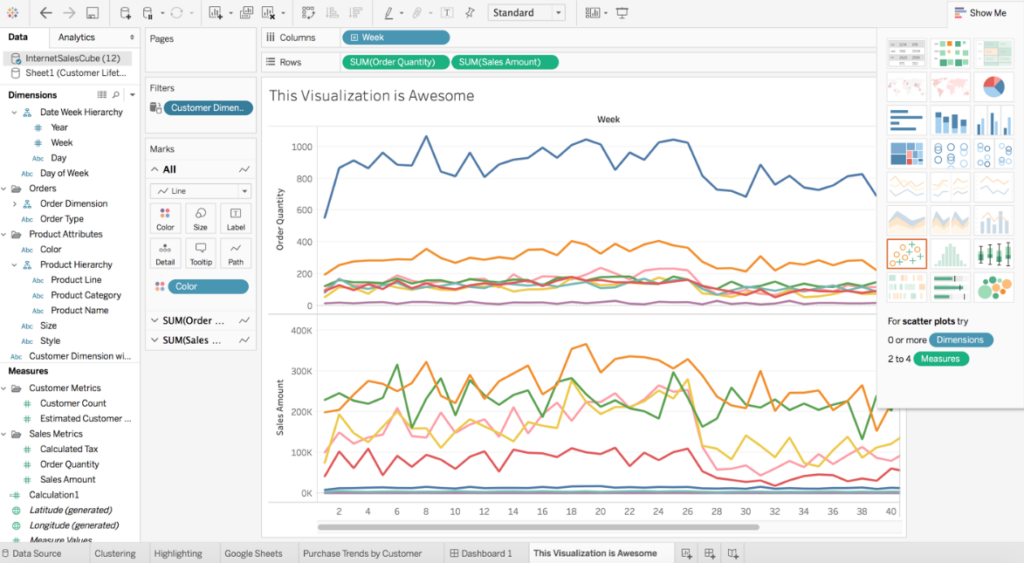
Tableau stands as a powerhouse in the realm of data visualization, allowing users to turn raw data into intuitive, interactive visualizations and dashboards. Its prowess in providing an immersive data exploration experience underscores its acclaim as the best tool for interactive big data visualization.
Why I Picked Tableau:
In my quest to find the pinnacle of visualization tools, Tableau continually emerged at the forefront. I chose it not merely for its popularity but for its undeniable capability to breathe life into big data, offering visuals that both inform and engage. Its reputation for crafting interactive data stories solidified my belief that it truly is the best for this unique endeavor.
Standout Features & Integrations:
Tableau excels with its drag-and-drop functionality, enabling users, regardless of their technical expertise, to create intricate visualizations with ease. Its real-time data analytics and collaborative features provide a holistic view, ensuring that insights are not only insightful but also actionable.
Beyond these features, Tableau boasts integrations with a multitude of data sources, from traditional databases to cloud services.
Pricing:
From $70/user/month (billed annually)
Pros:
- Intuitive drag-and-drop interface facilitates easy visualization creation
- Real-time analytics ensure timely insights
- Extensive integration capabilities with varied data sources
Cons:
- May have a steeper learning curve for beginners compared to other platforms
- Licensing costs can accumulate for larger teams
- Some advanced features may require scripting knowledge
7. Microsoft SQL Server - Best for relational database management
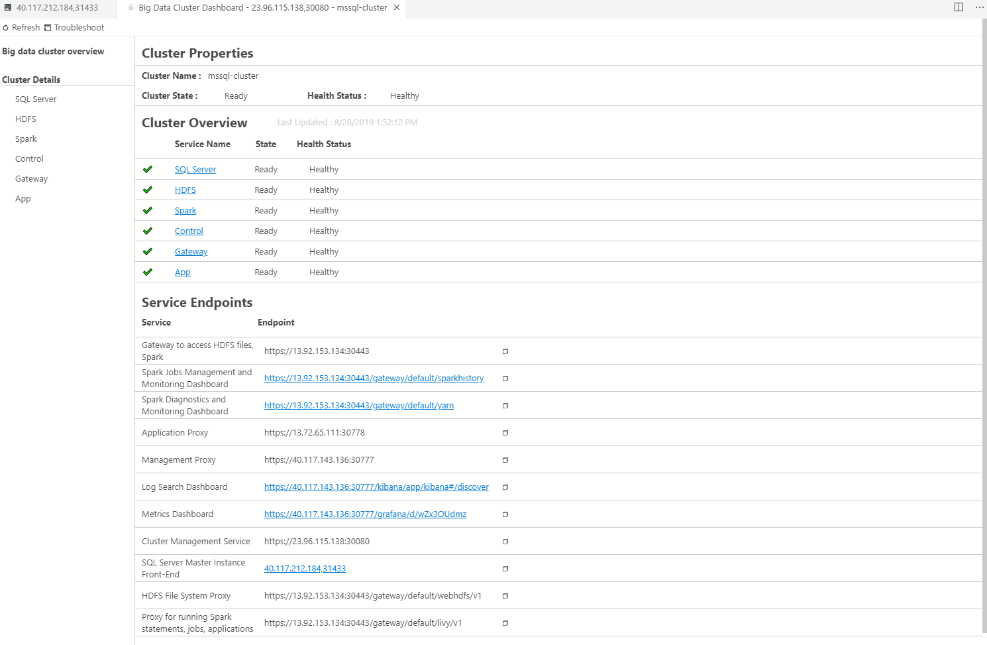
Microsoft SQL Server is a venerable product in the database domain, offering comprehensive tools for the storage, retrieval, and management of structured data. Its robust performance and reliability in handling relational databases make it a clear choice for organizations seeking effective relational database management.
Why I Picked Microsoft SQL Server:
Throughout my comparisons, I was drawn to Microsoft SQL Server primarily because of its consistency in delivering top-tier database solutions. I chose it for its deep integration with other Microsoft products, which streamlines many operational processes. Moreover, its historical track record and continuous advancements in relational database management techniques rendered it unparalleled in this domain.
Standout Features & Integrations:
Microsoft SQL Server is renowned for its high performance and security features, ensuring data remains both accessible and protected. Additionally, its Always On Availability Groups provide high availability and disaster recovery capabilities. Integration-wise, it syncs with other Microsoft products like Azure, Power BI, and Microsoft Office, fostering a harmonious data ecosystem.
Pricing:
From $25/user/month (billed annually)
Pros:
- Sturdy security features ensure data protection
- High availability with disaster recovery options through Always On Availability Groups
- Smooth integration with a wide range of Microsoft products
Cons:
- Might pose challenges for beginners unfamiliar with Microsoft's ecosystem
- Licensing model can be complex for some enterprises
- Resource-intensive, necessitating strong server hardware
8. Google Cloud BigQuery - Best for serverless and highly-scalable data warehousing
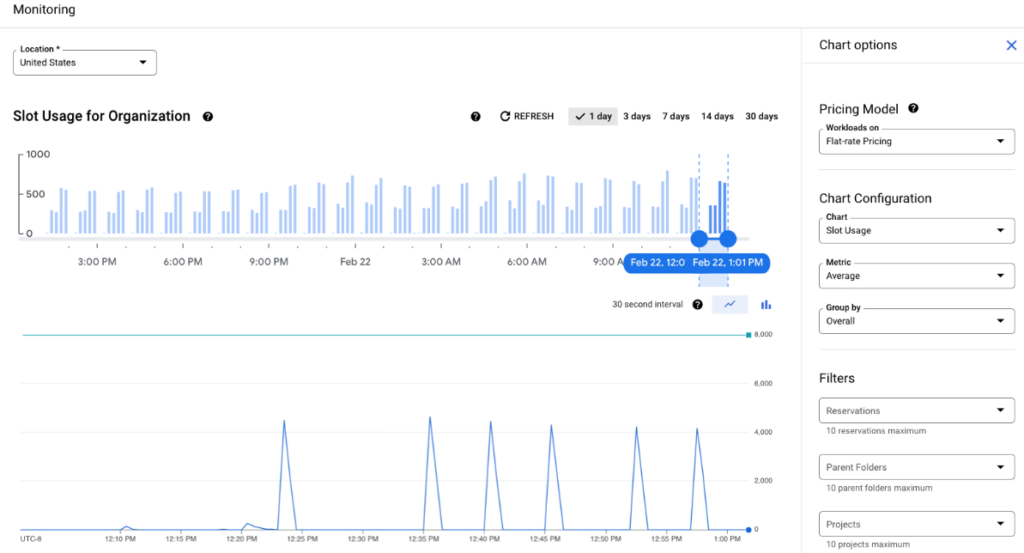
Google Cloud BigQuery provides a multi-cloud solution tailored for analyzing vast datasets in real-time. Its serverless architecture ensures scalability and simplicity, making it especially adept for those aiming to leverage data warehousing without the complexities of server management.
Why I Picked Google Cloud BigQuery:
After a rigorous assessment of various tools, I determined that BigQuery's serverless approach and its robust scaling mechanisms set it apart. I chose this tool because it eliminates the server management hassle while still providing a power-packed performance.
For businesses aiming to scale their data warehousing needs without diving deep into server intricacies, BigQuery is arguably the best choice.
Standout Features & Integrations:
BigQuery's real-time analytics capability enables businesses to make data-driven decisions promptly. Its automatic backup and easy-to-restore options ensure data safety and continuity. Integration-wise, BigQuery ties well with various Google Cloud services such as Dataflow, Pub/Sub, and AI Platform, giving users a holistic cloud experience.
Pricing:
From $20/user/month
Pros:
- Serverless nature provides hands-free scalability
- Real-time analytics empowers timely business decisions
- Easy integration with other Google Cloud services
Cons:
- Can become pricey for very high query volumes
- Learning curve for those unfamiliar with Google Cloud Platform
- Certain complex queries might demand optimization for cost and performance
9. Apache Flink - Best for real-time data processing and analytics
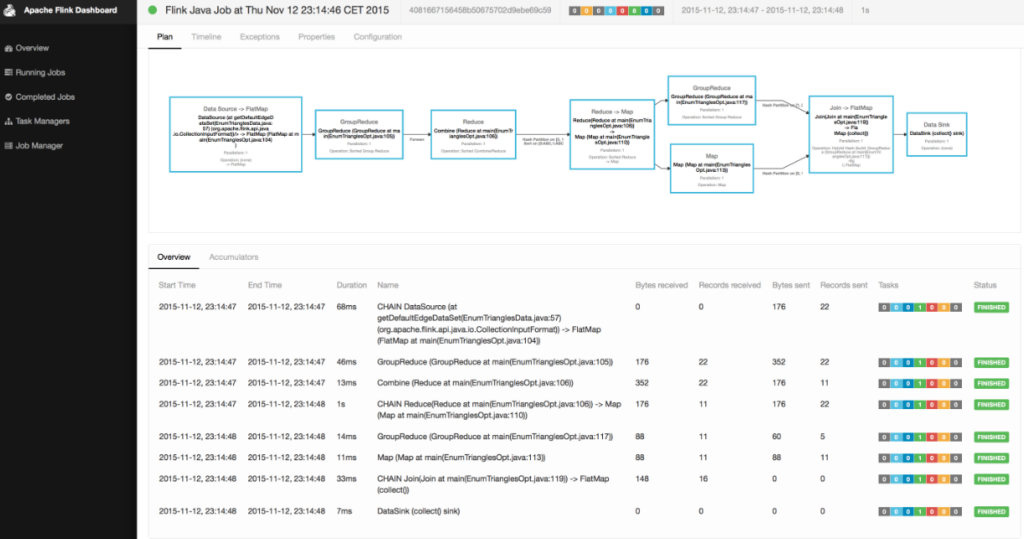
Apache Flink stands as a powerful, open-source stream processing framework. With its prowess in real-time data processing and analytics, it facilitates turning vast streams of data into actionable insights swiftly and efficiently.
Why I Picked Apache Flink:
In the process of determining the ideal tool for real-time analytics, Apache Flink consistently emerged at the forefront of my comparisons. Its distinctive stream-processing capabilities combined with its proven performance in complex data ecosystems made my decision clear.
For organizations that prioritize real-time insights and dynamic responses to data, Apache Flink presents an optimal solution.
Standout Features & Integrations:
Apache Flink boasts a fault-tolerant streaming model, ensuring data reliability and consistency. It supports event time processing, providing accurate results even when data arrives out of order. Flink integrates smoothly with popular data storage systems like Apache Kafka, Apache Cassandra, and AWS S3, offering flexibility in data sources.
Pricing:
Pricing upon request
Pros:
- Strong fault tolerance ensures data integrity during the processing
- Efficient event time processing handles out-of-order data with precision
- Rich integration capabilities allow connection with various data storage systems
Cons:
- Setup and configuration might require a steep learning curve for newcomers
- Scalability, while robust, may demand intricate tuning in massive-scale deployments
- As an open-source tool, commercial support options might be limited
10. Apache Kafka - Best for real-time data streaming platforms
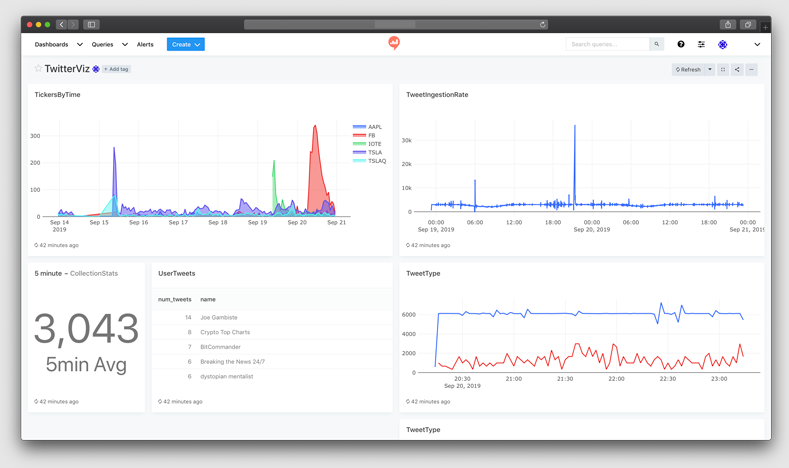
Apache Kafka is an open-source stream-processing software platform that functions as a real-time data streaming powerhouse. Its primary role revolves around managing and distributing streaming data across multiple sources, making it instrumental for organizations that demand real-time data insights.
Why I Picked Apache Kafka:
When selecting a tool centered around real-time data streaming, Apache Kafka frequently emerged as the leader in my evaluations. What differentiates Kafka is its robust architecture, its ability to process millions of events per second, and its vast adoption across industries.
My judgment aligns with the industry's, and I firmly believe Kafka excels as a choice for real-time data streaming platforms.
Standout Features & Integrations:
Apache Kafka's ability to durably store massive streams of records in a fault-tolerant manner is commendable. It is designed to allow applications to process, as well as analyze, data in real time. Furthermore, Kafka integrates efficiently with many third-party systems, prominently including Apache Spark, Apache Flink, and various data storage solutions.
Pricing:
Pricing upon request
Pros:
- Offers high throughput for both publishing and subscribing
- Provides durability and built-in redundancy for fault tolerance
- Supports integration with a myriad of third-party systems
Cons:
- The initial setup and tuning can be intricate for beginners
- Managing a Kafka cluster as it scales may introduce complexities
- Dealing with backpressure requires additional configurations and tooling
11. Apache Spark - Best for large-scale data processing tasks
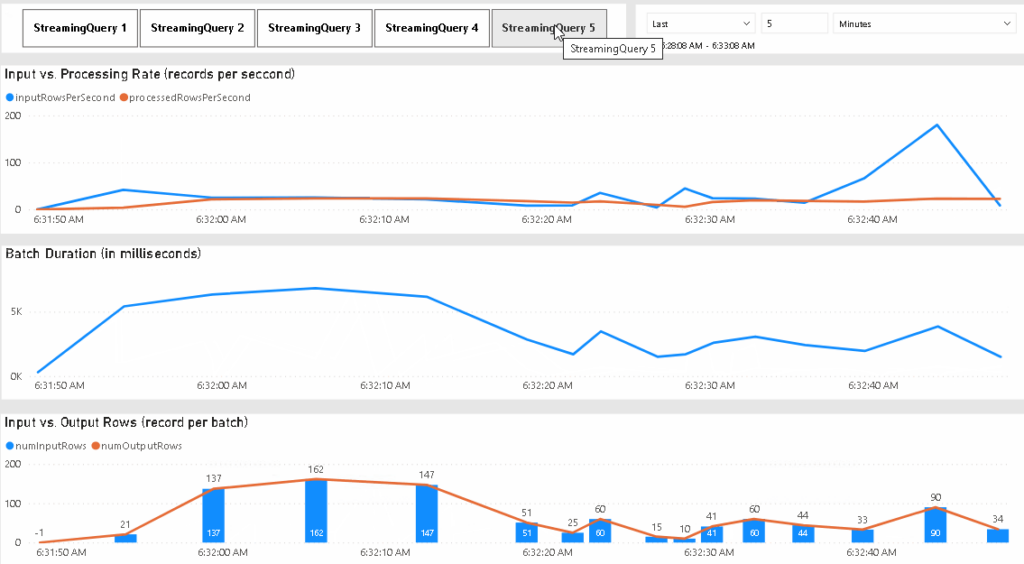
Apache Spark is an open-source distributed computing system tailored to handle a vast range of data processing tasks with a focus on speed and ease of use. The platform caters to organizations needing to process enormous datasets, justifying its aptness for large-scale data processing tasks.
Why I Picked Apache Spark:
When I sought a framework to manage mammoth data processing tasks, Apache Spark consistently proved to be a prominent choice. Its distinction lies in its in-memory computation capabilities, which considerably expedite large-scale data tasks. Given its proven efficiency and wide-scale industry adoption, I'm convinced that Spark is the top pick for large-scale data processing endeavors.
Standout Features & Integrations:
Apache Spark offers impressive features like in-memory processing which helps in reducing the time data spends on disks. Additionally, it boasts a unified platform, allowing users to combine SQL, streaming, and complex analytics. Spark’s ability to integrate with popular data storage tools, like HDFS and Apache Cassandra, and data processing tools like Apache Kafka, makes it versatile for diverse tasks.
Pricing:
Pricing upon request
Pros:
- Unparalleled speed due to in-memory processing
- Versatility in handling various tasks like batch processing, machine learning, and graph processing
- A rich set of developer libraries and APIs to extend its capabilities
Cons:
- May require significant memory for certain tasks, leading to higher operational costs
- The learning curve can be steep for newcomers
- Configuring and tuning Spark for optimal performance might be challenging
12. IBM Big Data Analytics - Best for enterprise-level data analysis and insights
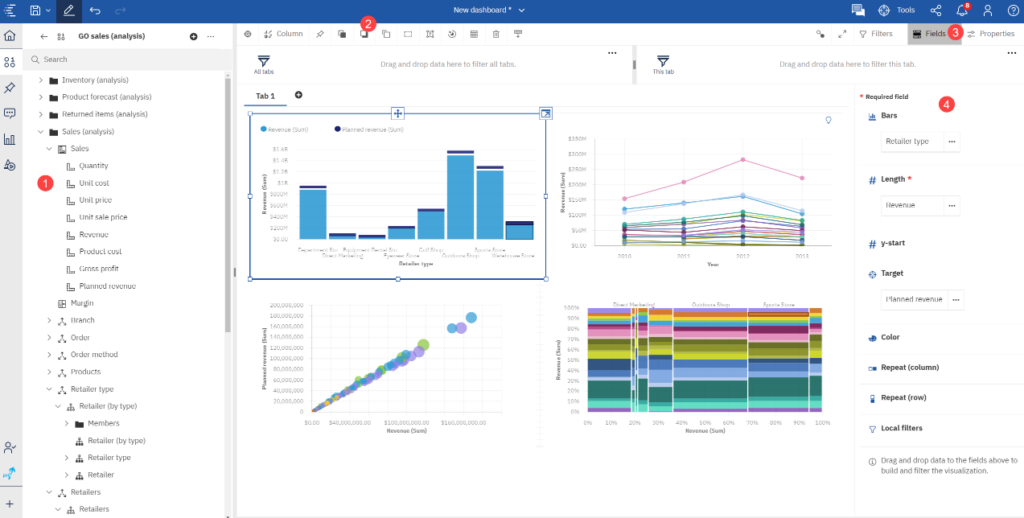
IBM Big Data Analytics is an integrated platform provided by IBM that is tailored to empower enterprises with the tools they need to derive actionable insights from their vast data repositories. With its sophisticated capabilities tailored to enterprises, it's the perfect fit for those seeking a comprehensive solution for data analysis and insights at a larger scale.
Why I Picked IBM Big Data Analytics:
When I delved into tools for enterprise-level data analysis, IBM Big Data Analytics emerged as a strong contender. I chose this platform due to its reputation for reliability and the vast suite of features it offers tailored for larger corporations.
This robust tool, combined with IBM's legacy in the tech industry, assured me that it is ideally suited for enterprise-level data analysis and insights.
Standout Features & Integrations:
IBM Big Data Analytics boasts a plethora of tools that allow for advanced predictive analytics, ranging from data exploration to machine learning. It incorporates AI-driven insights to make predictions more accurate and integrates with IBM's other data solutions.
Additionally, its compatibility with tools like Hadoop and Spark, as well as integration capabilities with many third-party platforms, further improves its value proposition.
Pricing:
Pricing upon request
Pros:
- Comprehensive suite of analytics tools that cater to a variety of needs
- Robust integration capabilities with both IBM and third-party solutions
- Reliable support and resources available given IBM’s reputation
Cons:
- Can be complex for those unfamiliar with IBM's ecosystem
- Deployment and setup might be more involved compared to other tools
- Cost may be prohibitive for smaller organizations
Other Noteworthy Big Data Software
Below is a list of additional big data software that I shortlisted but did not make it to the top 12. They are definitely worth checking out.
- Oracle Database - Good for robust enterprise data management
- Alteryx - Good for data blending and advanced analytics
- Databricks Lakehouse - Good for combining data lakes and data warehouses
- Azure Databricks - Good for cloud-native Apache Spark integration
- Snowflake - Good for scalable cloud data warehousing
- SAS Visual Analytics Software & Analysis Tools - Good for interactive data visualization
- Minitab - Good for statistical analysis and process improvement
- Apache HBase - Good for large-scale columnar storage
- Apache Cassandra - Good for high-availability distributed databases
- MongoDB - Good for flexible NoSQL document storage
- Apache Hive - Good for SQL-like querying on Hadoop data
- Impala - Good for real-time querying in Hadoop
- Apache NiFi - Good for automating and managing data flows
- FlinkML - Good for scalable machine learning on streaming data
Selection Criteria for Choosing the Best Big Data Software
In this evaluation, I specifically sought software that can provide uncompromised data integrity, flexibility in operations, and intuitive interfaces. Based on my hands-on experience, here's a breakdown of the primary criteria that should guide your decision-making.
Core Functionality
- Data Storage: Store structured and unstructured data without compromising on speed or accessibility.
- Query Execution: Rapidly fetch or modify data based on specific conditions.
- Backup and Recovery: Regularly save data and facilitate quick recovery in case of loss or corruption.
- Data Replication: Duplicate data across different environments or servers for load balancing or redundancy.
- Data Integrity Checks: Ensure data remains accurate, consistent, and reliable throughout its lifecycle.
Key Features
- Data Security: Features like encryption, access controls, and audit logs to protect sensitive data.
- Scalability: The capability to handle growing amounts of data without sacrificing performance.
- Indexing and Searching: Swift search functionalities aided by effective indexing mechanisms.
- Data Relationships: Facilitate relations between tables or datasets, enhancing the richness of data queries.
- Migration Capabilities: Efficiently move data between different database systems or versions.
Usability
- Graphical User Interface (GUI): A user-friendly visual environment to manage, query, and manipulate data without extensive coding.
- Drag-and-Drop Query Builder: Simplify query creation by allowing users to visually structure their requests.
- Role-Based Views: An interface that adjusts based on user roles, presenting only relevant tools and data models.
- Comprehensive Documentation: A rich library of resources, from beginner guides to advanced troubleshooting tips, to aid users at all expertise levels.
- Reliable Support: A team that's available and knowledgeable, offering guidance during setup, troubleshooting, or even during complex operations.
Most Common Questions Regarding Big Data Software
What are the benefits of using big data software?
Using big data software offers numerous advantages, such as:
- Efficient Data Management: Handle vast volumes of structured and unstructured data easily.
- Advanced Analytics: Unlock insights from data using machine learning, AI, and statistical algorithms.
- Real-time Processing: Analyze and act on data science in real-time, making decision-making faster and more accurate.
- Scalability: These tools can scale to accommodate growing data without compromising performance.
- Data Integration: Integrate data from multiple sources, ensuring a comprehensive view of information.
How much do these big data tools typically cost?
The cost of big data software varies based on its features, scalability, and brand. Many tools offer a tiered pricing model, which means you pay based on the functionalities you need and the amount of data you handle.
What are the common pricing models for these tools?
Most Big Data tools follow one of these pricing models:
- Subscription-based: Monthly or yearly fees for access to the software.
- Pay-as-you-go: Pricing is based on data usage or the number of queries run.
- Freemium: Basic features are free, with advanced functionalities available for a fee.
What is the typical range of pricing for big data software?
Prices can range anywhere from $10/month for basic functionalities to thousands of dollars a month for enterprise-level solutions. It largely depends on the tool's capabilities and the scale at which a business operates.
Which is the cheapest big data software available?
There are several budget-friendly options available in the market, but tools like Apache Hadoop and Apache Spark offer open-source solutions, which can be more cost-effective for businesses with limited budgets.
Which big data software is the most expensive?
Enterprise-level solutions like Oracle's Big Data Suite or IBM's Big Data Platform tend to be on the pricier side, especially when used by large corporations with massive data volumes.
Are there any free big data software options available?
Yes, there are open-source big data software options available, like Apache Hadoop, Apache Hive, and Apache Cassandra. These tools are free to use, but businesses might incur costs for setting up, managing, or customizing them.
Why is there such a variation in pricing among these tools?
The pricing difference often arises due to the variety of features offered, the software's scalability, support services, brand reputation, and the targeted audience (small businesses vs. large enterprises).
Other Data Management Software Reviews
- Database Design Tools
- Data Masking Software
- Data Integration Tools
- Desktop Database Software
- Data Replication Software
Summary
Navigating the world of big data software can initially seem overwhelming due to the sheer number of options available and the varied functionalities they offer. However, when armed with the right knowledge and criteria, pinpointing the best fit for your specific needs becomes a clearer and more manageable task.
Key takeaways:
- Define Your Core Needs: Before diving into the sea of options, clearly define what you expect the software to accomplish for you. By focusing on core functionality tailored to your specific use case, you can filter out unsuitable options.
- Consider Usability and Support: Beyond just features, the design and user experience can significantly impact the efficiency and effectiveness of any software. Ensure the tool you choose aligns well with your team's proficiency and offers adequate customer support.
- Understand Pricing Models: With various pricing structures in place across different tools, understand what you're paying for and ensure it aligns with your budget. Remember, the most expensive option isn't always the best; it's about value for money and fit for purpose.
What Do You Think?
I strive to keep my guide comprehensive and up-to-date, but the world of big data software is vast and ever-evolving. If you've come across a noteworthy tool that I haven't mentioned, or if you have firsthand experience with a new software making waves in the industry, I'd love to hear from you. Please share your suggestions and insights in the comments below or reach out to me directly. Let's work together to make this guide even better for everyone.