Best DataOps Tools Shortlist
Here’s my shortlist of the best DataOps tools:
Our one-on-one guidance will help you find the perfect fit.
Broken data pipelines. Failed ETL jobs. Compliance audits that turn into fire drills. If you’ve dealt with any of these, you already know why DataOps tools are becoming essential. Managing data across dev, staging, and production environments is hard enough—doing it manually or across siloed teams makes it nearly impossible.
I’ve worked with data engineers and product teams to implement and evaluate DataOps platforms in high-growth SaaS environments. I’ve tested tools that promise to solve everything and found the ones that actually deliver: from automated quality checks and pipeline monitoring to lineage tracking and role-based access controls.
In this guide, I’ll share the DataOps tools that stand out—so your team can spend less time fixing and more time delivering reliable, compliant, and high-quality data.
Why Trust Our Software Reviews
We’ve been testing and reviewing SaaS development software since 2023. As tech experts ourselves, we know how critical and difficult it is to make the right decision when selecting software. We invest in deep research to help our audience make better software purchasing decisions.
We’ve tested more than 2,000 tools for different SaaS development use cases and written over 1,000 comprehensive software reviews. Learn how we stay transparent & check out our software review methodology.
Best DataOps Tools Summary
This comparison chart summarizes pricing details for my top DataOps tools selections to help you find the best one for your budget and business needs.
Tool | Best For | Trial Info | Price | ||
---|---|---|---|---|---|
1 | Best for data privacy management | Free trial + free demo available | From $150/month (billed annually) | Website | |
2 | Best for large-scale data analytics | Not available | From $99/user/month | Website | |
3 | Best for data prep automation | 30-day free trial | From $433/user/month (billed annually) | Website | |
4 | Best for data testing and validation | Free demo available | Pricing upon request | Website | |
5 | Best for dataops orchestration | Free demo available | Free to use | Website | |
6 | Best for enterprise data integration | Free consultation available | Pricing upon request | Website | |
7 | Best for search and data analytics | 14-day free trial | From $16/user/month (billed annually) | Website | |
8 | Best for data quality monitoring | Not available | From $500/user/month (billed annually) | Website | |
9 | Best for SQL-like querying | Not available | Free to use | Website | |
10 | Best for hybrid data integration | Free demo available | From $1/user/hour | Website |
-
Docker
This is an aggregated rating for this tool including ratings from Crozdesk users and ratings from other sites.4.6 -
Pulumi
This is an aggregated rating for this tool including ratings from Crozdesk users and ratings from other sites.4.8 -
GitHub Actions
Visit Website
Best DataOps Tools Reviews
Below are my detailed summaries of the best DataOps tools that made it onto my shortlist. My reviews offer a detailed look at the key features, pros & cons, integrations, and ideal use cases of each tool to help you find the best one for you.
Ketch is a data privacy management software tailored for businesses focusing on compliance and user privacy. It serves legal, marketing, and technology professionals by offering tools like consent management and data mapping.
Why I picked Ketch: It focuses on ensuring compliance with regulations like GDPR and CCPA. Ketch automates privacy processes, including data subject request (DSR) automation and risk assessments, which saves your team time. You also get customizable privacy banners to enhance customer relationships. Its ability to integrate with over 1,000 systems adds flexibility, making it a great choice for privacy-focused businesses.
Standout features & integrations:
Features include customizable privacy templates, risk assessment tools, and automated workflows. You can use Ketch to easily manage consent and data subject requests, improving productivity by reducing manual tasks. The platform also offers a user-friendly interface that supports quick setup and onboarding.
Integrations include Salesforce, HubSpot, Marketo, Microsoft Dynamics, Google Analytics, Adobe Experience Cloud, Amazon Web Services, Oracle, SAP, and Workday.
Pros and cons
Pros:
- Quick setup and onboarding
- Supports over 1,000 integrations
- Enhances customer relationships
Cons:
- Requires ongoing regulatory updates
- Limited customization options
New Product Updates from Ketch
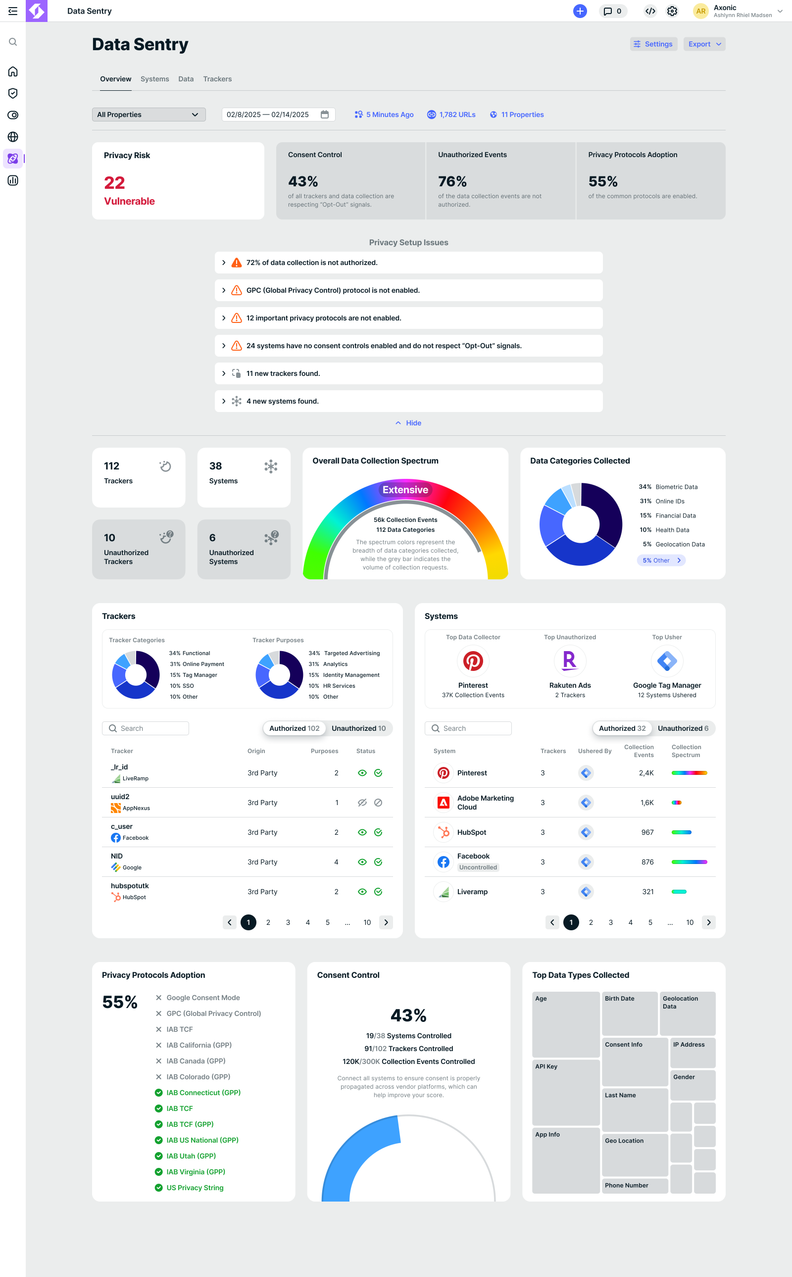
Introducing Data Sentry: Safeguarding Privacy and Compliance
Ketch introduced Data Sentry, a tool that scans network traffic to identify privacy risks, ensuring data collection aligns with consent policies by detecting third-party data transfers and validating opt-out compliance. For more details, visit Ketch Blog.
Databricks is a data and AI platform designed for enterprises, catering to data engineers, scientists, and analysts. It offers data processing, analytics, and machine learning functionalities.
Why I picked Databricks: It excels in large-scale data analytics, offering collaborative notebooks and Delta Lake for data reliability. Your team can benefit from its advanced machine learning tools, enhancing analytical capabilities. The platform's robust support for Apache Spark ensures efficient big data processing. Flexible integration with major cloud providers like AWS, Azure, and Google Cloud further enhances its appeal for large-scale operations.
Standout features & integrations:
Features include collaborative notebooks that foster teamwork and innovation. Delta Lake ensures data reliability and consistency, which is crucial for big data tasks. The platform's machine learning capabilities let your team build and deploy models efficiently.
Integrations include Apache Spark, AWS, Azure, Google Cloud, Tableau, Power BI, Snowflake, MongoDB, Looker, and Qlik.
Pros and cons
Pros:
- Advanced machine learning tools
- Supports big data processing
- Flexible cloud integrations
Cons:
- Occasional performance issues
- Complex pricing structure
Alteryx is a data analytics platform that helps users prepare, blend, and analyze data from various sources. It offers both desktop and cloud-based solutions, catering to professionals who need to automate data workflows and derive insights efficiently.
Why I picked Alteryx: You can automate repetitive data preparation tasks using Alteryx's drag-and-drop interface. It provides over 300 no-code and low-code tools, allowing your team to clean, transform, and enrich data without writing code. The platform supports both structured and unstructured data, enabling you to handle diverse datasets. Additionally, Alteryx offers AI-driven recommendations to optimize your workflows.
Standout features & integrations:
Features include tools for geospatial analytics, enabling your team to perform location-based analysis. You can also utilize built-in machine learning capabilities to create predictive models without extensive coding knowledge. Furthermore, Alteryx supports real-time data processing, allowing you to make timely decisions based on current data.
Integrations include connections with Salesforce, Snowflake, Amazon Redshift, Google BigQuery, Microsoft Azure, Tableau, Power BI, Oracle, SAP, and Databricks.
Pros and cons
Pros:
- Handles both structured and unstructured data
- Automates complex data preparation tasks
- Offers AI-driven workflow recommendations
Cons:
- Requires separate licensing for some features
- Limited data visualization capabilities
RightData is a data quality and testing platform that caters to data engineers and analysts. It provides data validation, testing, and reconciliation functionalities, ensuring data accuracy and reliability.
Why I picked RightData: It specializes in data testing and validation, offering features like automated data reconciliation and validation rules. Your team can automate testing processes to save time and reduce errors. The platform's intuitive interface simplifies managing complex data projects. Customizable dashboards offer insights into data quality, making it easier to maintain accuracy.
Standout features & integrations:
Features include customizable validation rules that let you tailor data checks to meet your specific needs. Automated reconciliation processes help you identify discrepancies quickly. You can also use its intuitive interface to manage and monitor data testing efficiently.
Integrations include Salesforce, SAP, Oracle, SQL Server, Hadoop, AWS, Azure, Google Cloud, Informatica, and Teradata.
Pros and cons
Pros:
- Intuitive user interface
- Customizable validation rules
- Automated reconciliation processes
Cons:
- May require technical expertise
- Limited customization for complex needs
DataKitchen is a DataOps platform designed for data engineers and analysts, providing orchestration and automation of data workflows. It helps streamline and manage data operations, ensuring efficiency and accuracy.
Why I picked DataKitchen: It excels in dataops orchestration, offering features like automated workflows and data versioning. Your team can use its environment management capabilities to maintain consistency across different stages. The platform's error tracking and alerting system ensures quick resolution of issues. DataKitchen's support for agile methodologies helps your team adapt to changes swiftly.
Standout features & integrations:
Features include automated data testing, which ensures data quality at every stage. You can use its collaborative workspace to improve teamwork and communication. The platform also offers deployment automation, reducing the time and effort required for releases.
Integrations include AWS, Azure, Google Cloud, Snowflake, Databricks, Tableau, Power BI, Apache Kafka, Apache Airflow, and Jenkins.
Pros and cons
Pros:
- Automated data testing
- Efficient error tracking
- Supports agile methodologies
Cons:
- Customization options can be limited
- Requires learning curve adjustment
IBM’s DataOps platform is designed for large enterprises, catering to IT professionals and data engineers. It focuses on data integration, governance, and quality, ensuring that your data operations are efficient and reliable.
Why I picked IBM: It excels in enterprise data integration, offering tools like data cataloging and governance. You can automate workflows, which helps in maintaining data quality across your organization. The platform's data lineage feature provides transparency, allowing you to track data sources and transformations. Its scalability suits large enterprises needing to manage vast amounts of data efficiently.
Standout features & integrations:
Features include data cataloging that helps you organize your data assets. The platform's governance tools ensure compliance with data regulations. Automated data quality checks help maintain the integrity of your datasets.
Integrations include IBM Cloud, AWS, Azure, Google Cloud, Hadoop, Apache Spark, Oracle, SAP, Salesforce, and Tableau.
Pros and cons
Pros:
- Automated quality checks
- Supports large-scale data operations
- Comprehensive data governance tools
Cons:
- Limited customization options
- May have a steep learning curve
Elastic is an open-source search and analytics engine that provides real-time insights from your data. Its core function is to store, search, and analyze vast amounts of data quickly and in near real-time. This makes Elastic exceptionally proficient for real-time search and data analytics tasks.
Why I Picked Elastic:
I chose Elastic due to its high-speed search capabilities and its ability to handle massive datasets effectively. Its powerful search features, coupled with its real-time analytics capabilities, make Elastic stand out from other tools.
Based on these capabilities, I determined Elastic to be the best tool for real-time search and data analytics.
Standout Features and Integrations:
Features include full-text search, distributed search, and real-time analytics. Its real-time, multi-level aggregation functionality helps users explore and analyze their data more intuitively.
Integrations include numerous data collection and visualization tools, including Logstash for centralized logging and Kibana for data visualization.
Pros and cons
Pros:
- Flexible with data formats
- Can handle large datasets
- Provides fast and efficient search results
Cons:
- While the basic Elastic stack is free, advanced features come with a cost
- Configuring and tuning Elastic for specific use cases can be complex
Badook is a data quality monitoring tool designed for data engineers and analysts. It focuses on ensuring data accuracy and reliability by identifying and addressing data quality issues in real-time.
Why I picked Badook: It excels in data quality monitoring, providing features like automated anomaly detection and data validation. Your team can use its real-time dashboards to monitor data health continuously. The platform's customizable alerts ensure you’re notified of issues promptly. With its user-friendly interface, you can easily set up and maintain data quality checks.
Standout features & integrations:
Features include customizable dashboards that let you visualize data quality metrics effectively. Automated reporting tools help you track data health over time. The platform also offers detailed audit trails, which provide insights into data changes and quality issues.
Integrations include AWS, Azure, Google Cloud, Snowflake, Databricks, Apache Kafka, Tableau, Power BI, MongoDB, and Apache Hive.
Pros and cons
Pros:
- User-friendly interface
- Customizable alerts for data issues
- Real-time monitoring dashboards
Cons:
- Customization options may be limited
- Can be resource-intensive
Apache Hive is a data warehousing solution that primarily serves data analysts and engineers. It allows for querying and managing large datasets stored in distributed storage.
Why I picked Apache Hive: It offers SQL-like querying, which is ideal for users familiar with SQL. The tool's integration with Hadoop makes it suitable for analyzing large data sets efficiently. Apache Hive supports various data formats, giving your team flexibility in data handling. Its ability to handle complex queries with ease makes it a reliable choice for data-driven businesses.
Standout features & integrations:
Features include a query language similar to SQL, which simplifies learning and adoption. It supports indexing to improve query performance, making data retrieval faster. Apache Hive also offers partitioning, which helps manage and query large datasets efficiently.
Integrations include Hadoop, HDFS, HBase, Amazon S3, Azure Blob Storage, Google Cloud Storage, Apache Spark, Apache Pig, Apache Tez, and Apache Ranger.
Pros and cons
Pros:
- Handles large datasets efficiently
- Supports complex data queries
- SQL-like query language
Cons:
- Performance varies with data size
- Not ideal for real-time analytics
Azure Data Factory is a cloud-based data integration service for data engineers and analysts. It facilitates the creation, scheduling, and orchestration of data workflows, supporting both cloud and on-premises data sources.
Why I picked Azure Data Factory: It excels in hybrid data integration, allowing your team to connect diverse data environments. The platform's drag-and-drop interface simplifies the creation of complex workflows. You can use its built-in scheduling to automate data movement, reducing manual tasks. Real-time monitoring capabilities provide insights into data processes, enhancing operational efficiency.
Standout features & integrations:
Features include an intuitive drag-and-drop interface for designing data pipelines. Built-in scheduling tools let you automate data workflows, saving time and effort. Real-time monitoring provides visibility into data operations, helping you identify and resolve issues quickly.
Integrations include Azure Blob Storage, Azure SQL Database, Azure Synapse Analytics, Amazon S3, Google BigQuery, Oracle, SAP, Salesforce, Teradata, and IBM Db2.
Pros and cons
Pros:
- Real-time monitoring capabilities
- Supports diverse data environments
- Easy-to-use drag-and-drop interface
Cons:
- Limited offline functionality
- Initial setup complexity
Other DataOps Tools
Here are some additional DataOps tools options that didn’t make it onto my shortlist, but are still worth checking out:
- Apache NiFi
For real-time data flow management
- StreamSets
For real-time data movement
- HighByte
For industrial data integration
- Talend
For open-source data integration
- Cloudera
For hybrid cloud data solutions
- Druid
For real-time analytics
- Perfect
For monitoring data workflows
- Beam
For real-time data processing
- Hadoop
For distributed storage and processing
- Composable
For data orchestration and automation
- Airflow
For orchestrating complex computational workflows
- Kafka
For high-throughput data streaming
- Trifecta
For data cleaning and preparation
- Kubernetes
For container orchestration
- dbt
For data modeling and transformation
- Atlan
For collaborative workspace
- Jupyter
For interactive data science
- Snowflake
For a fully-managed cloud data platform
- Qubole
For cloud-native big data activation platform
DataOps Tools Selection Criteria
When selecting the best DataOps tools to include in this list, I considered common buyer needs and pain points like data integration challenges and maintaining data quality. I also used the following framework to keep my evaluation structured and fair:
Core Functionality (25% of total score)
To be considered for inclusion in this list, each solution had to fulfill these common use cases:
- Data integration and transformation
- Data quality management
- Workflow automation
- Real-time data processing
- Compliance and governance
Additional Standout Features (25% of total score)
To help further narrow down the competition, I also looked for unique features, such as:
- Customizable data pipelines
- Advanced data lineage tracking
- Machine learning capabilities
- Real-time anomaly detection
- Scalable architecture
Usability (10% of total score)
To get a sense of the usability of each system, I considered the following:
- Intuitive user interface
- Ease of navigation
- Customizable dashboards
- Minimal learning curve
- Responsive design
Onboarding (10% of total score)
To evaluate the onboarding experience for each platform, I considered the following:
- Availability of training videos
- Interactive product tours
- Comprehensive documentation
- Access to webinars
- Supportive chatbots
Customer Support (10% of total score)
To assess each software provider’s customer support services, I considered the following:
- 24/7 support availability
- Multi-channel support options
- Knowledgeable support staff
- Quick response times
- Availability of community forums
Value For Money (10% of total score)
To evaluate the value for money of each platform, I considered the following:
- Competitive pricing
- Flexible subscription plans
- Transparent pricing structure
- Availability of free trials
- Cost-benefit ratio
Customer Reviews (10% of total score)
To get a sense of overall customer satisfaction, I considered the following when reading customer reviews:
- Overall satisfaction ratings
- Commonly mentioned pain points
- Praise for specific features
- Feedback on customer support
- Frequency of updates and improvements
How to Choose DataOps Tools
It’s easy to get bogged down in long feature lists and complex pricing structures. To help you stay focused as you work through your unique software selection process, here’s a checklist of factors to keep in mind:
Factor | What to Consider |
Scalability | Ensure the tool can grow with your data needs. Look for features that support large datasets and increasing user numbers. |
Integrations | Verify compatibility with existing systems. Check if it supports APIs, databases, and third-party applications you already use. |
Customizability | Consider how well the tool adapts to your processes. Look for options to tailor dashboards, workflows, and reports. |
Ease of Use | Evaluate the user interface and learning curve. Your team should find it intuitive and quick to adopt. |
Budget | Assess the total cost, including hidden fees. Compare pricing plans to ensure they fit your financial constraints. |
Security Safeguards | Analyze the tool's security features. Ensure it complies with data protection standards and offers encryption. |
Support Services | Check availability of customer support. Look for 24/7 assistance, documentation, and training resources. |
Performance | Test the tool's speed and reliability. It should handle data processing efficiently without lags. |
Trends in DataOps Tools
In my research, I sourced countless product updates, press releases, and release logs from different DataOps tools vendors. Here are some of the emerging trends I’m keeping an eye on:
- AI-Driven Insights: More tools are using AI to provide predictive analytics and insights. This helps teams anticipate issues and optimize processes. Vendors like Alteryx are incorporating AI to enhance data analysis capabilities.
- Real-Time Data Processing: The demand for real-time data processing is growing. It allows businesses to make quicker decisions based on up-to-date information. Tools like StreamSets focus on real-time data movement to meet this need.
- Data Governance Enhancements: Companies are focusing on improved data governance features. This ensures compliance with regulations and enhances data quality. IBM's DataOps platform offers strong governance tools to address these concerns.
- Self-Service Capabilities: There's a shift towards empowering non-technical users with self-service options. This makes data tasks more accessible across teams. Talend has been adding self-service features to simplify data integration.
- Cloud-Native Architectures: More tools are being designed for cloud environments. This offers greater flexibility and scalability for businesses managing large datasets. Azure Data Factory exemplifies this trend with its cloud-native data integration services.
What Are DataOps Tools?
DataOps tools are software solutions designed to automate and enhance data management processes. Data engineers, analysts, and IT professionals typically use these tools to improve data quality, governance, and integration.
Real-time processing, AI-driven insights, and self-service capabilities help with optimizing workflows, ensuring compliance, and making data accessible. Combined with robust data observability tools, these solutions increase efficiency and accuracy in data operations, benefiting businesses by enabling better decision-making.
Features of DataOps Tools
When selecting DataOps tools, keep an eye out for the following key features:
- Real-time data processing: This enables immediate data analysis, allowing businesses to make quick decisions based on current information.
- AI-driven insights: These provide predictive analytics, helping teams anticipate issues and optimize data processes.
- Data governance: Ensures compliance with regulations and maintains data quality across operations.
- Self-service capabilities: Empowers non-technical users to manage data tasks independently, increasing accessibility.
- Cloud-native architecture: Offers flexibility and scalability for managing large datasets in cloud environments.
- Automated workflows: Streamlines data management tasks, reducing manual effort and minimizing errors.
- Customizable dashboards: Allows users to tailor data visualization to their specific needs, enhancing understanding.
- Data lineage tracking: Provides transparency by showing data's origin and transformation, ensuring accuracy.
- Integration support: Facilitates smooth data flow between various systems and applications, enhancing compatibility.
- Error handling: Quickly identifies and resolves issues, minimizing disruptions in data processing.
Benefits of DataOps Tools
Implementing DataOps tools provides several benefits for your team and your business. Here are a few you can look forward to:
- Improved data quality: Automated workflows and data governance ensure your data is accurate and reliable.
- Faster decision-making: Real-time data processing allows your team to act on the latest insights without delay.
- Enhanced compliance: Strong data governance features help your business meet regulatory requirements effortlessly.
- Increased accessibility: Self-service capabilities let non-technical users handle data tasks, broadening access.
- Scalability: Cloud-native architecture supports your growing data needs without compromising performance.
- Reduced manual effort: Automated error handling and workflows minimize repetitive tasks, freeing up your team's time.
- Better collaboration: Customizable dashboards and real-time insights foster teamwork and informed decision-making.
Costs and Pricing of DataOps Tools
Selecting DataOps tools requires an understanding of the various pricing models and plans available. Costs vary based on features, team size, add-ons, and more. The table below summarizes common plans, their average prices, and typical features included in DataOps tools solutions:
Plan Comparison Table for DataOps Tools
Plan Type | Average Price | Common Features |
Free Plan | $0 | Basic data integration, limited storage, and community support. |
Personal Plan | $10-$30/user/month | Data integration, basic analytics, limited automation, and email support. |
Business Plan | $50-$100/user/month | Advanced analytics, automation, data governance, and priority support. |
Enterprise Plan | $150-$300/user/month | Custom integrations, full automation, enhanced security, and dedicated support. |
DataOps Tools: FAQs
Here are some answers to common questions about DataOps tools:
What are some important considerations for implementing DataOps?
When implementing DataOps, you should build cross-functional teams to enhance collaboration between data engineers, developers, and analysts. Choose the right tools that fit your needs and establish clear processes for your team. Automate repetitive tasks to save time, and continuously monitor and iterate on your processes.
What are the three pipelines of DataOps?
DataOps typically involves three pipelines: Production, Development, and Environment. Each serves a specific purpose in managing data flows. Production pipelines handle live data, development pipelines focus on testing and building, and environment pipelines manage configurations. Understanding these helps in orchestrating efficient workflows.
How is DataOps different from DevOps?
While DevOps focuses on unifying development and operations teams for software delivery, DataOps aims to break down silos between data producers and consumers. The goal is to make data more accessible and valuable. DataOps emphasizes collaboration across data teams to enhance data management and usage.
What are the key benefits of automating DataOps tasks?
Automating DataOps tasks can significantly enhance efficiency by reducing manual effort. It ensures consistency in data processes and helps you maintain data quality. Automation also allows your team to focus on more strategic tasks, leading to improved productivity and faster decision-making.
How do DataOps tools support data governance?
DataOps tools offer features that help enforce data governance policies. They provide visibility into data processes, ensuring compliance with regulations. By tracking data lineage and offering audit trails, these tools help maintain data integrity and transparency across your organization.
Why is real-time data processing important in DataOps?
Real-time data processing allows your team to access the most current data, enabling quick decision-making. It supports dynamic business needs by providing up-to-date insights. This capability is crucial for industries where timely data is key to staying competitive and responsive to market changes.
What's Next?
Boost your SaaS growth and leadership skills.
Subscribe to our newsletter for the latest insights from CTOs and aspiring tech leaders.
We'll help you scale smarter and lead stronger with guides, resources, and strategies from top experts!