Data trust has become more important than ever in the era of AI. With the rapidly increasing reliance on data to power gen AI and other AI applications, organizations must evaluate data management strategies and frameworks to best support this new and evolving landscape.
Data trust ensures the reliability and accuracy of an organization's data. It goes beyond investing in individual tools for observability, cataloging, or governance—it's about establishing a comprehensive framework that guarantees trustworthy data throughout the entire value chain.
For example, a retail company leveraging AI for personalized marketing must ensure its customer data is accurate and up-to-date to avoid targeting the wrong demographics with inappropriate products.
The Challenge of Legacy Systems
The main challenge is that traditional technologies often fall short when handling the scale and intricacy of modern data ecosystems. These legacy approaches struggle with data quality, observability, cataloging, and governance - critical aspects frequently siloed and disjointed in legacy systems. This fragmentation can lead to data inconsistencies, governance challenges, and increased risk.
For instance, a financial institution like Equifax using outdated systems might face challenges in maintaining consistent data quality across different departments, leading to errors in compliance reporting or financial forecasting.
The Data Trust Maturity Framework
To address these challenges and build a solid foundation for AI success, organizations should consider adopting a data trust maturity framework, which provides a roadmap for evolving from the early stages of data management to a sophisticated, scalable data governance ecosystem.
This roadmap includes:
- Early-stage organizations focus on metadata management and manual testing of key assets. While reactive, this stage is crucial for establishing the groundwork for more advanced practices. For example, a startup may begin by manually auditing its customer data to ensure accuracy before launching its AI-driven recommendation engine.
You can join the modern data revolution by (1) implementing controls to help ensure the quality of source data, (2) establishing tools and processes for shared (semantic) data understanding early in your organization’s data flows, and (3) democratizing data work by adopting low code / no code and generative AI tools for data consumption.

- Aware and Growing Stage Here, an organization’s focus shifts to a more proactive approach, introducing a business glossary, tracking data lineage, assigning data ownership, and implementing basic data observability. This step is essential for building a common data language and fostering collaboration between data teams and business stakeholders. A mid-sized company might start implementing a business glossary to standardize terms and definitions across its various departments, ensuring everyone is on the same page when analyzing sales data.
- Scale Stage Introduces advanced practices such as PII masking, formal governance structures, comprehensive infrastructure coverage, data contracts, and the creation of data products and domains. At this stage, data is treated as a product, with deliberate design and maintenance aimed at meeting the needs of its users. A large corporation might establish formal data governance structures to manage sensitive customer information across multiple global markets, ensuring compliance with local regulations and enhancing trust with customers.
Speaking from my experience helping federal agencies and organizations better manage their data within and without the context of emerging tech like AI, the success of data trust in the age of AI hinges on high-quality data, but achieving and maintaining this quality presents significant challenges.
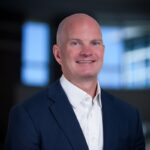
Implementing such a unified data trust maturity framework is key to overcoming the limitations of conventional technologies and effectively navigating the complexities of AI and digital transformation. Such a framework should address several interconnected components:
- Data Discovery and Metadata Management
- Data Domain Classification and Prioritization
- Data Observability for Reliability and Performance Monitoring
- Enhanced Collaboration and Data Contracts
- Data Governance Strategies
By consolidating these functions into a single platform, organizations can enjoy seamless integration, reduced vendor dependency, and enhanced communication across their data landscape.
A healthcare organization using a unified platform can streamline data sharing between different departments, reducing the risk of data breaches and improving patient outcomes through better-informed clinical decisions.
Good data collection should be done with intentionality. What are the key questions that you are asking AI to help you solve? What data are needed to answer those questions? By designing business processes that are data-driven from start to finish, organizations can help to make data collection a more routine part of their day-to-day operations. When end users use systems properly, the data they generate is more accurate, and the insights generated from predictive models or used to enhance prompts to LLMs can actually drive meaningful business value.
At the end of the day, AI models amount to little more than a science experiment if their outputs aren’t used to make better decisions. To ensure that this happens, organizations must prioritize driving trust — and ultimately, adoption — of these tools.
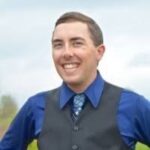
Overcoming GenAI Challenges
One of the most significant challenges facing organizations today is the increasing complexity of GenAI models. These models are often trained on massive datasets, making it difficult to understand how they arrive at their conclusions. This lack of transparency can make it difficult to trust the results of GenAI models.
Mike Finley, CTO and Co-founder of AnswerRocket, highlights this challenge: "Language models introduce a whole new attack surface for data governance policy. Sending data away to a model unlocks massive value but also exposes new threat vectors. Enterprises are divided on this issue, with traditionally conservative teams like IT and Legal holding back cautiously while strategy teams hit the gas."
In addition to the challenges of transparency, GenAI models can also be susceptible to bias. If the data used to train a GenAI model is biased, the model will also be biased. This can lead to unfair or discriminatory outcomes.
To address these challenges, organizations must be aware of the limitations of GenAI models and take steps to mitigate the risks. This includes implementing strong data governance practices, ensuring that data is unbiased, and being transparent about the limitations of GenAI models.
It's important to note, however, that implementing a data trust framework is as much about internal cultural change as it is about tools and processes; it requires time, dedication, and a mindset shift across the organization.
As Ted Vial points out, building trust with AI starts with the data used to train it.
AI is quickly becoming the new search engine, creating a significant opportunity for the spread of false information. AI companies need to build trust with their users, starting first and foremost with the data they use to generate responses.
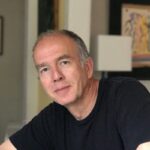
Data Trust as a Competitive Advantage in AI
As the new era of AI continues to grow and evolve, data trust is a cornerstone of successful AI-driven strategies. The journey from traditional systems to a robust, scalable, and trustworthy data ecosystem demands cultural shifts, investments in governance, and a forward-thinking approach to technology.
In this new era, clean, reliable data will distinguish market leaders from the rest. Organizations that place data trust at the heart of their AI initiatives will be well-positioned to innovate faster, deliver more personalized experiences, and secure long-term growth. By focusing on data trust today, you're laying the groundwork for tomorrow's AI-powered success.
Subscribe to The CTO Club newsletter for more insights on AI, data trust, and the future of tech leadership.